Understanding Decision Support Systems in Agriculture
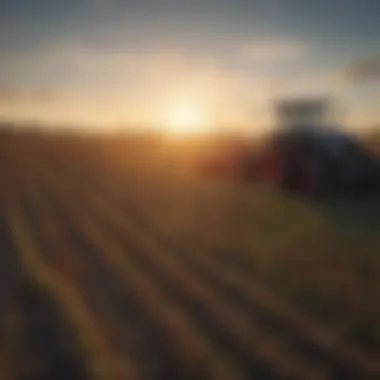
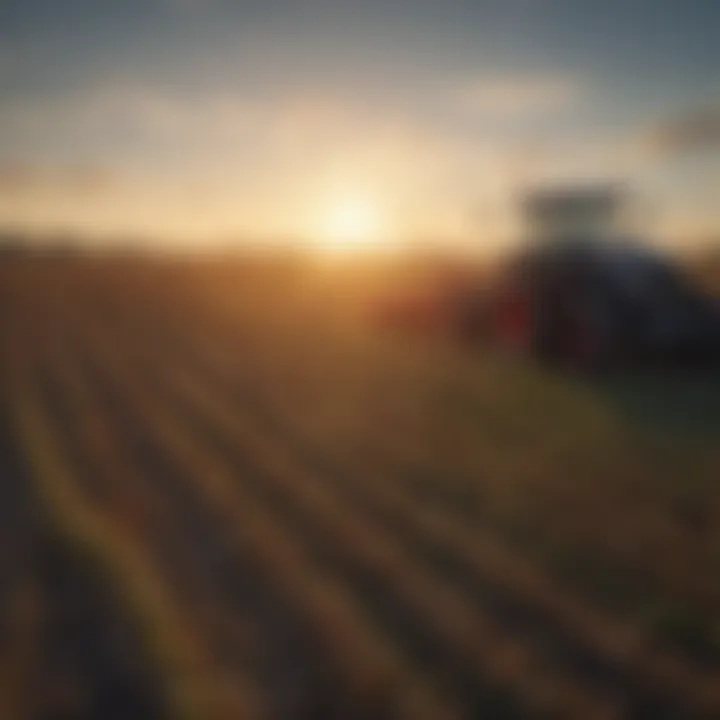
Intro
Decision Support Systems (DSS) have emerged as critical tools in the agricultural sector. They enable farmers and agronomists to make informed decisions based on data analysis. These systems integrate various components including data management, algorithm selection, and user interfaces. By utilizing DSS, stakeholders can improve crop management, enhance sustainability practices, and optimize resource use. As the agricultural landscape becomes more complex due to climate change and population growth, the reliance on effective decision-making systems becomes even more important.
Overview of the Topic
Definition and Importance
A Decision Support System in agriculture is a computer-based program that analyzes data to aid decision-making. DSS can synthesize information from field sensors, weather data, and historical records to offer actionable insights. The importance of DSS lies in their ability to reduce uncertainty in farming operations and enhance productivity. These systems support precision agriculture, helping to increase yield while minimizing costs and environmental impact.
Current Trends
Recent trends reveal the growing integration of advanced technologies in decision support systems. Artificial Intelligence and machine learning algorithms are increasingly being employed to improve data analysis capabilities. These technologies provide predictive analytics, enabling farmers to anticipate challenges before they arise. Furthermore, the use of geographic information systems (GIS) is becoming widespread in DSS, allowing for nuanced spatial analysis.
Key Techniques and Practices
Step-by-Step Guide
- Identify Objectives: Determine the specific decisions that need support, such as irrigation scheduling or pest management.
- Data Collection: Gather relevant data from sensors, satellite imagery, and weather stations.
- Data Integration: Merge different data sources to create a comprehensive dataset.
- Select Algorithms: Choose appropriate algorithms for analysis based on the objectives.
- Run Analysis: Execute the chosen models on the integrated dataset.
- Output Interpretation: Translate the results into actionable recommendations.
Tools and Equipment Needed
To effectively utilize DSS in agriculture, certain tools are essential:
- Sensors: For real-time data collection, including soil moisture and temperature sensors.
- Software Platforms: Such as Cropio or Trimble Ag Software, which provide interfaces for data management and analysis.
- Cloud Computing: Enables the storage and processing of large datasets remotely.
Challenges and Solutions
Common Obstacles
Despite their potential, DSS face several challenges:
- Data Accuracy: Poor quality or incomplete data can lead to ineffective recommendations.
- User Adoption: Farmers may be hesitant to adopt new technologies due to a lack of familiarity.
- System Integration: Different data sources may not communicate effectively with each other.
Innovative Solutions
To combat these challenges, several solutions can be implemented:
- Data Cleaning Protocols: Establishing standard procedures for verifying data accuracy before input.
- Training Programs: Offering workshops and resources to aid user understanding of DSS functionality.
- Open Standards: Developing interoperable systems that facilitate better communication across platforms.
The successful implementation of DSS in agriculture hinges on overcoming challenges related to data quality and system integration.
Prologue to Decision Support Systems
Decision Support Systems (DSS) have emerged as a pivotal tool for farmers and agronomists seeking to enhance their decision-making processes. In the context of agriculture, these systems provide actionable insights that can lead to improved productivity and sustainability. They help to analyze vast amounts of data, forecasting outcomes and offering recommendations that can lead to better management practices.
The integration of technology into agriculture has significantly transformed traditional farming practices. However, the effectiveness of such transformation largely depends on the availability and utilization of relevant information. This is where DSS comes into play — bridging the gap between data collection and meaningful decision-making. It enables users to leverage data in ways that were not previously possible, emphasizing its importance in modern agricultural practices.
Definition of Decision Support Systems
A Decision Support System is a computerized system that supports decision-making activities. In agriculture, it collects, processes, and analyzes data to assist users in making informed choices. A DSS typically includes data management systems, model management systems, and a user interface that allows users to interact with the system efficiently.
The core of a DSS lies in its ability to process complex data and present it in a way that is understandable and actionable. For example, by using weather data, soil conditions, and crop health indicators, a DSS can suggest optimal planting times or recommend resource allocation strategies.
Historical Context and Evolution
The concept of Decision Support Systems dates back several decades. Initially, DSS were used primarily in corporate environments to analyze business data. The evolution of computing technology and data analysis tools has expanded the scope of DSS into various fields, including agriculture.
As agriculture began incorporating technology, the need for sophisticated data analysis grew. Early applications focused on basic data analysis, but advancements in artificial intelligence and big data have propelled DSS to new heights. Today, they play a crucial role in precision agriculture, offering insights based on real-time data and predictive analytics.
Importance of DSS in Agriculture
The significance of Decision Support Systems in agriculture cannot be overstated. They enable farmers to:
- Enhance Productivity: By providing insights into optimal planting strategies, yield forecasts, and resource management, DSS helps to boost the overall yield.
- Reduce Costs: Effective resource allocation can minimize waste and unnecessary expenditure.
- Facilitate Sustainable Practices: DSS aids in making environmentally-friendly decisions by analyzing the impact of agricultural practices on the ecosystem.
- Improve Risk Management: With predictive modeling, farmers can prepare for adverse conditions like pests, weather changes, or market fluctuations, thereby reducing the risk of crop failures.
In summary, Decision Support Systems represent a significant evolution in agricultural practices, underscoring the importance of data-driven decision-making in enhancing farm productivity and sustainability.
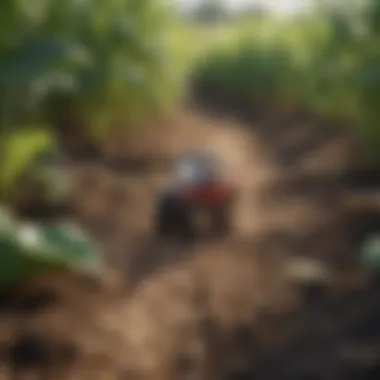
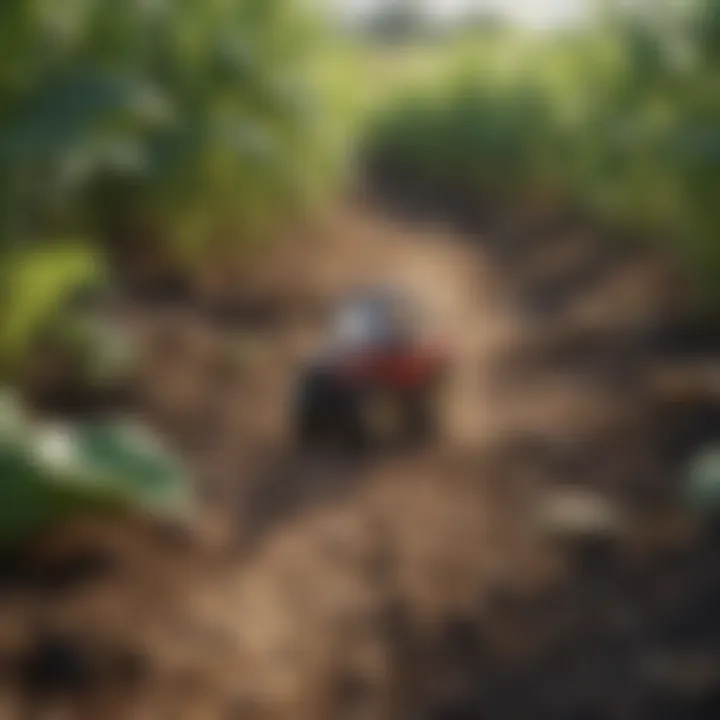
Key Components of DSS
Understanding the key components of Decision Support Systems (DSS) is essential for anyone interested in the intersection of technology and agriculture. These components serve as the foundation for effective decision-making processes in farming. Each aspect impacts how information is collected, analyzed, and presented to the end-user, ultimately influencing farm management outcomes.
Data Management Systems
Data Management Systems are central to DSS. They focus on the collection, storage, and retrieval of data. In agriculture, this data can range from soil conditions to weather forecasts. Effective data management ensures that all relevant data is accurately captured and structured in a way that is easily accessible.
A well-organized data management system allows for timely and informed decisions. Without high-quality data, the potential of DSS diminishes. Farmers can utilize historical data trends to predict yields or assess risks related to pest infestations. Thus, the reliability of data management systems directly correlates with the accuracy of predicted outcomes.
Key considerations for data management include:
- Data Source Integrity: Ensuring consistency and reliability of data from all sources.
- Storage Solutions: Utilizing cloud systems or local databases that can grow and adapt to growing data needs.
Model Management
Model Management involves the algorithms and simulations that DSS use to interpret and analyze data. These models provide the necessary tools for scenario analysis, helping farmers to plan for different eventualities, from crop diseases to market fluctuations.
The effectiveness of a Decision Support System hinges on the models' validity and reliability. If a model fails to reflect real-world conditions accurately, it can lead to poor decision-making. Farmers must understand the limitations of these models and ensure they are grounded in local expertise and agricultural practices.
Critical aspects of Model Management include:
- Model Selection: Choosing models that are relevant to specific agricultural scenarios.
- Validation and Testing: Regularly assessing models against real-data outcomes to ensure accuracy.
User Interface Design
User Interface Design plays a crucial role in the usability of DSS. A well-designed interface enables farmers to navigate complex data insights easily. Intuitive interface design allows users to interact with the system without extensive training. This eases the adoption barrier for many potential users.
Factors to consider in User Interface Design include:
- Accessibility: The interface should be usable across various devices like smartphones, tablets, and computers.
- Visualization: Effective graphical representations of data will help users quickly comprehend information and trends.
- User Feedback: Implementing feedback loops to improve the system based on user experiences can enhance the overall function.
In summary, the key components of DSS—Data Management Systems, Model Management, and User Interface Design—must work cohesively for effective decision-making in agriculture. Each component contributes uniquely to the system's effectiveness and is crucial for the modern farmer aiming for optimization and sustainability.
Functionality and Operation
The functionality and operation of Decision Support Systems (DSS) play a crucial role in their effectiveness within the agricultural landscape. These systems are designed to assist farmers and agronomists in making informed decisions grounded in data-driven insights. The complexity of agricultural decision-making necessitates a DSS that integrates both data collection and analytics, scenario simulation, and robust support algorithms. Understanding these elements can help improve farming practices, resource management, and overall yield.
Data Collection and Analysis
Data collection is the first step in the operation of any DSS. In the agricultural context, this includes various forms of data: soil characteristics, weather patterns, crop health, and market trends. Sensors and IoT devices are often employed to gather real-time data, ensuring accuracy and relevance. The analysis of this information provides actionable insights.
- Importance of Data Quality: High-quality data directly affects the reliability of recommendations made by the DSS. Farmers must ensure that the data is accurate, timely, and comprehensive to optimize their decision-making process.
- Data Sources: Sources may include satellite imagery, climatic data from meteorological bodies, and even historical farming data. Integrating multiple sources helps build a more complete picture of the farming environment.
- Benefits: Through effective data analysis, farmers can identify trends and patterns that inform their decisions on planting times, resource allocation, and pest management strategies.
Scenario Simulation and Predictive Modeling
Scenario simulation and predictive modeling are vital features of advanced DSS. These functions allow users to explore various what-if scenarios that can influence agricultural planning. By simulating different conditions—like changes in weather, pest outbreaks, or market fluctuations—farmers can better prepare for uncertainties.
- Modeling Techniques: Techniques may include statistical models and machine learning algorithms, which forecast the impact of specific variables on crop yields or resource usage.
- Decision Impact Evaluation: Users can evaluate the potential impacts of various decisions before actually implementing them. This foresight can minimize risks associated with farming.
- Real-Time Adjustments: With predictive modeling, adjustments can be made in real-time based on changing conditions—like using data to shift irrigation methods or crop types based on weather predictions.
Decision Support Algorithms
The core of any DSS lies in its decision support algorithms. These algorithms process the collected data and simulate scenarios to provide actionable insights tailored to specific user needs. Choosing the right algorithms is critical for effective decision support.
- Types of Algorithms: Common types include optimization algorithms, heuristics, and artificial intelligence methods that analyze vast datasets efficiently. They allow farmers to optimize for maximum yield or minimum costs.
- User-Centric Design: Algorithms must also be user-friendly, delivering insights in an understandable format. This ensures the farmers can act on the information without requiring advanced technical knowledge.
- Integration with DSS: Effective integration of algorithms with the data management and user interface ensures seamless operation and enhances user experience.
"Effective decision support in agriculture hinges on data quality, timely analysis, and user-friendly algorithms."
In summary, the functionality and operation of DSS encompass a range of activities from data collection to decision support algorithms, each contributing to enhanced agricultural productivity. Understanding these components allows farmers to harness the full potential of technology in their practices.
Applications of DSS in Agriculture
The applications of Decision Support Systems (DSS) in agriculture are manifold and significant. They enable farmers and agronomists to make informed decisions based on comprehensive data analysis, ultimately aiding in enhancing productivity and sustainability. Understanding these applications goes beyond recognizing their features; it involves appreciating the critical advantages they offer in modern agricultural practices.
Crop Management Strategies
Crop management is fundamental to successful farming. DSS provides frameworks for planning and executing better crop management strategies. With the incorporation of data related to soil health, weather patterns, and crop yield, DSS solutions can analyze multiple scenarios and suggest planning rotations, planting schedules, and harvest timings accordingly.
For instance, through predictive modeling, farmers can assess the potential outcomes of different planting strategies. DSS can use real-time data to inform decisions about which crops to plant based on the current market conditions, thereby maximizing profits. Additionally, these systems can help manage pest control and irrigation, offering solutions tailored to specific farm conditions. It's crucial for farmers to leverage these strategies to boost yield while minimizing costs.
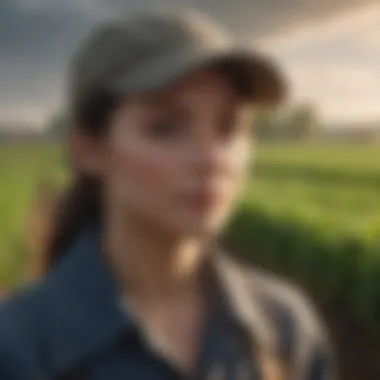
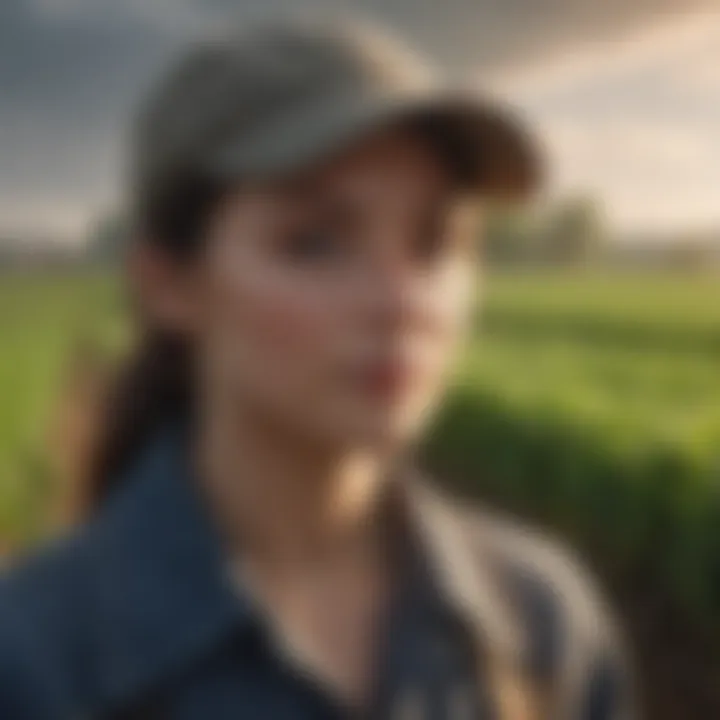
Resource Optimization
Resource optimization is another critical aspect of DSS in agriculture. As resources like water and fertilizers become increasingly scarce and costly, efficient management becomes paramount. Decision Support Systems facilitate the optimal use of these resources by employing advanced algorithms that analyze usage data against crops' actual needs.
By utilizing DSS, farmers can:
- Monitor resource usage: Track how and where resources are being utilized in real-time.
- Analyze efficiency: Identify inefficiencies in resource application to adjust practices accordingly.
- Save costs: Reduce waste and lower expenses through precise application recommendations.
The outcomes of such optimizations can lead to significant cost savings and improved environmental impacts. Farmers can utilize data-driven insights to apply fertilizers only when necessary, thus preventing runoff and promoting soil health.
Sustainable Practices Integration
Sustainability is not just a buzzword in agriculture; it is a necessity for the future of food production. DSS plays a pivotal role in integrating sustainable practices into farming operations. These systems allow for evaluation of the long-term impacts of agricultural practices on the environment.
By facilitating:
- Soil conservation strategies: DSS can guide farmers in implementing practices that protect against erosion.
- Biodiversity checks: They can evaluate the effects of crop rotation on local ecosystems and promote diverse planting.
- Climate change adaptation: DSS aids in forecasting climatic impacts, enabling farmers to adapt practices proactively.
In this way, farm operations can evolve toward more sustainable models without sacrificing productivity. Such integration not only benefits the land but also enhances marketability, as consumers increasingly seek sustainably produced goods.
The effective use of Decision Support Systems can redefine agricultural practices, leading to enhanced efficiency and sustainability in farming.
In summary, the applications of DSS in agriculture present a transformative approach to modern challenges faced by farmers. From maximizing crop yield to optimizing resource use and promoting sustainability, DSS offers tools that are indispensable for contemporary agricultural operations.
Challenges of Implementing DSS
In the agricultural sector, Decision Support Systems (DSS) represent a powerful tool for optimizing farm management and enhancing productivity. However, implementing these systems is not without challenges. This section focuses on three main challenges: data quality issues, system integration difficulties, and user adoption and training. Each of these elements plays a crucial role in the successful deployment of DSS in agriculture, highlighting the importance of addressing these challenges effectively.
Data Quality Issues
Data quality is critical for the efficacy of any Decision Support System. Inaccurate, incomplete, or outdated data can lead to poor decision-making. Farmers rely on DSS to provide real-time insights based on available data. If this data is flawed, the recommendations generated by the system may not reflect the actual conditions of the farm.
Key factors contributing to data quality issues include:
- Inconsistent Data Sources: Different data collection methods may yield discrepancies. For example, satellite imagery might not align with ground truth data collected by farmers.
- Human Error: Manual data entry and management processes are prone to mistakes—incorrect entries can compromise the entire system's reliability.
- Outdated Information: Agricultural conditions change rapidly; therefore, systems that do not update in real-time can miss critical trends.
To mitigate these issues, it is essential to establish rigorous data validation and preprocessing protocols. Additionally, investing in reliable sensors and automated data collection methods can significantly increase data integrity.
System Integration Difficulties
The integration of Decision Support Systems with existing farm management tools can pose significant challenges. Farms often utilize multiple systems for various functions—like irrigation management, crop monitoring, and financial management. Achieving seamless communication among these tools is imperative for a holistic approach to farm management.
Challenges in system integration often include:
- Compatibility Issues: Different software platforms may not communicate effectively, hindering data flow and functionality.
- High Costs of Integration: Upgrading or replacing legacy systems can be expensive and time-consuming, and many farmers may be unwilling to take such risks.
- Vendor Lock-In: Some agricultural solutions may restrict interoperability with other systems, limiting farmers’ flexibility.
Effective integration requires a thorough understanding of existing systems and careful planning. Utilizing open standards and APIs can help overcome integration barriers, ensuring that systems work together harmoniously.
User Adoption and Training
Even with a well-designed DSS, its benefits cannot be realized unless users know how to utilize it effectively. Training and user adoption are vital components of a successful DSS implementation. Many farmers may be hesitant to adapt to new technologies, which can limit the overall effectiveness of the system.
Factors impacting user adoption include:
- Lack of Technical Skills: Some farmers might not possess the necessary skills or knowledge to navigate complex systems, leading to frustration.
- Resistance to Change: Established routines and traditional methods can create resistance among farmers, as they may be skeptical about the benefits of new approaches.
- Insufficient Training Programs: Many training initiatives are inadequate in addressing farmers' specific needs, resulting in a lack of confidence in using the system.
To enhance user acceptance, it is essential to provide tailored training programs that consider the skill level and the operational context of the users. Additionally, support and feedback channels can help farmers feel more comfortable with adopting new technologies.
"To be successful in integrating Decision Support Systems, addressing data quality, integration challenges, and user acceptance is not optional but essential."
In summary, while Decision Support Systems have the potential to revolutionize agricultural practices, overcoming implementation challenges is crucial. A systematic approach focusing on improving data quality, enhancing integration capabilities, and fostering user understanding can unlock the full benefits of DSS in agriculture.
Case Studies of DSS in Agriculture
Case studies serve as a crucial element in comprehending the real-world impact of Decision Support Systems (DSS) in agriculture. They provide tangible evidence of success and failure, revealing the conditions under which DSS can thrive or struggle. By investigating these case studies, stakeholders can derive insights that inform future implementations and innovations.
Success Stories from the Field
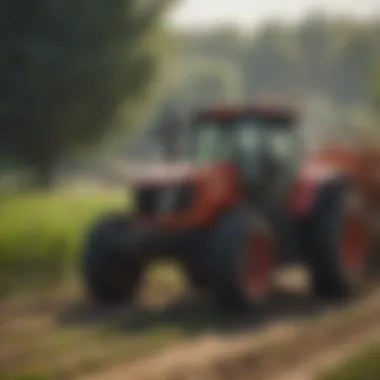
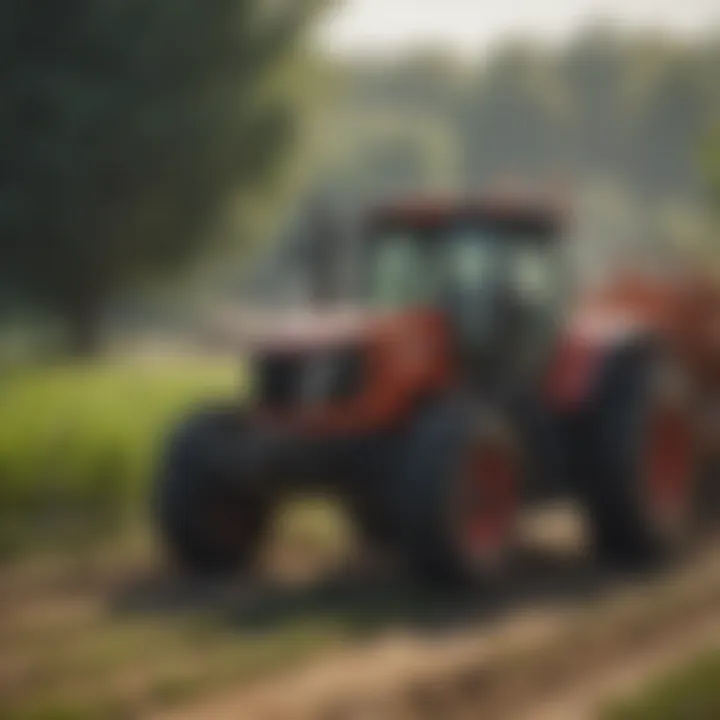
Many farmers and agronomists have successfully integrated DSS into their practices, enhancing productivity and sustainability. For instance, a prominent case in precision agriculture involves the use of Climate FieldView. This platform utilizes satellite imagery and data analytics to offer farmers actionable insights regarding their crops. As a result, farmers have reported increased yields and reduced resource waste.
Another example can be found in Ag Leader Technology, which has created decision support software specifically designed for nutrient management. They employ data to tailor fertilization plans that match the specific needs of crops under varying conditions. The implementation of such technologies demonstrates how DSS can lead to improved crop health and financial outcomes.
These cases highlight essential aspects of effectively leveraging DSS:
- Real-Time Data Use: Successful systems rely heavily on accurate, up-to-date data.
- User Engagement: When users are actively involved in the development and implementation phases, outcomes tend to be more favorable.
- Tailored Solutions: Customization of DSS-tools to fit specific farming needs can significantly enhance effectiveness.
"Innovations in Decision Support Systems offer the potential not only for increased efficiency but also for reduced environmental impact, which is essential in modern agriculture."
Lessons Learned from Failed Implementations
Not all attempts at implementing DSS in agriculture have been successful. Understanding the reasons behind these failures is just as important. A notable example is the deployment of a DSS in managing water resources in a drought-stricken area. The system faced resistance from local farmers who were either skeptical about its effectiveness or lacked the necessary technological skills to operate it. Consequently, the DSS was underutilized and failed to achieve its intended goals.
Several key considerations emerge from these failed implementations:
- Lack of User Training: Insufficient training leads to miscommunication and underutilization of DSS functionalities. Training programs should be comprehensive and ongoing.
- Poor Data Quality: Decisions based on inaccurate or incomplete data can severely undermine the reliability of the system.
- Inadequate Stakeholder Engagement: Successful implementation requires a buy-in from all stakeholders. Without involvement from the end-users, support can dwindle, leading to project failure.
Future Trends in DSS
In the rapidly evolving agricultural sector, the implementation of Decision Support Systems (DSS) is becoming increasingly crucial. Understanding the future trends in DSS is essential for farmers and agronomists looking to harness the full potential of technology in agriculture. Advances are shaping how decisions are made, optimizing resource usage, and enabling more sustainable practices. Among these trends, the integration with Internet of Things (IoT) and smart farming techniques plays a critical role alongside the advancements in artificial intelligence and machine learning applications.
Integration with IoT and Smart Farming
The integration of IoT devices with DSS is a transformative trend that empowers farmers to collect real-time data from their fields. Sensors installed in fields can monitor soil moisture, temperature, and crop health. This data is then sent to the DSS, which analyzes it to provide actionable insights.
- Real-Time Monitoring: IoT devices enable continuous tracking of various environmental factors affecting crop yield, resulting in timely decisions that could mitigate risks.
- Automated Responses: Smart farming practices allow systems to automatically respond to changing conditions. For instance, if soil moisture drops below a certain level, irrigation systems can activate without manual intervention.
- Data Management: Integrating IoT technology simplifies data collection processes, ensuring the DSS works with accurate and up-to-date information.
Farmers benefit from this integration through increased efficiency, reduced labor costs, and improved crop yield potential. The synergy between IoT and DSS fosters a more connected and informed approach to farm management.
Artificial Intelligence and Machine Learning Applications
Artificial intelligence and machine learning represent another pivotal area for the future of Decision Support Systems in agriculture. These technologies facilitate advanced data analysis and predictive modeling, enhancing decision-making capabilities.
- Predictive Analytics: Machine learning algorithms can analyze historical data to predict future outcomes, allowing farmers to plan proactively. For example, predicting pest outbreaks can help in preparing preventive measures before damage occurs.
- Yield Optimization: AI can analyze varied factors affecting crop yields. By understanding these interrelations, farmers can optimize their inputs and processes, ensuring better resource allocation.
- Personalized Advisory Services: With AI, DSS can provide tailored advice based on individual farm data, leading to more customized management strategies.
The adoption of artificial intelligence ensures that agricultural stakeholders are more equipped to face the challenges posed by changing climate conditions and market demands.
The Role of DSS in Decision Making
Decision Support Systems (DSS) play a pivotal role in refining the decision-making landscape in agriculture. These systems are not merely tools; they are critical frameworks that assist farmers and agronomists in navigating complex agricultural challenges. The significance of DSS extends beyond its basic functionalities, as it provides a comprehensive platform for integrating data, assessing risks, and improving overall farm management. The efficiency gained through DSS directly correlates to increased productivity and sustainability in agricultural operations.
Enhancing Efficiency and Productivity
DSS enhances efficiency in agricultural practices by streamlining processes and reducing time-intensive tasks. For instance, data analysis becomes faster and more accurate, allowing farmers to make informed choices promptly. With the integration of real-time data, such as weather forecasts and soil conditions, farmers can tailor their actions accordingly. This timely information helps in planning planting schedules, irrigation needs, and pest management, leading to optimal resource allocation.
Moreover, productivity benefits arise from detailed analytics provided by DSS. Farmers can track crop yields and make comparisons over time, identifying trends and areas for improvement. This analytical power equips farmers to make strategic decisions that maximize outputs while minimizing waste. In essence, the efficiency gained through DSS translates into better resource use, cost savings, and ultimately, higher profitability.
Facilitating Data-Driven Decisions
DSS facilitates data-driven decisions by aggregating relevant information from multiple sources. Farmers have access to extensive datasets, including historical yield data, market trends, and environmental factors. Such depth of information promotes informed decision-making founded on empirical evidence rather than intuition alone.
The systems often utilize advanced algorithms to analyze this data, offering insights into the potential outcomes of different decision paths. For instance, a farmer can evaluate the impact of changing crop varieties or altering fertilization practices based on comprehensive data analysis.
This data-centric approach empowers farmers to make decisions that align closely with current agricultural conditions and market demands. The result is a more agile response to challenges, increased resilience, and better long-term planning.
"A well-functioning Decision Support System can transform uncertainty into actionable insights, helping farmers make choices that are not only informed but also strategic."
As the agricultural landscape evolves, the implementation of DSS becomes crucial for adapting to the demands of modern farming. By leveraging these systems effectively, farmers can harness technology to enhance their decision-making processes.
Culmination
In this article, the discussion on Decision Support Systems (DSS) in agriculture provides a thorough overview of their significance in enhancing decision-making for farmers and agronomists. As agriculture faces increasing pressure from climate change, market demands, and resource constraints, DSS play a vital role in optimizing operations and improving outcomes.
Summary of Key Points
The key elements of DSS involve components such as data management, model management, and user interface design. These elements work collectively to facilitate data collection, analysis, and effective decision-making. The importance of applications like crop management, resource optimization, and integrating sustainable practices reflects the practical impacts DSS can have. Furthermore, the challenges in implementing these systems, such as data quality issues, integration difficulties, and user adoption barriers, underline the complexities involved in harnessing such technologies.
Implications for Future Research
Future research avenues must focus on enhancing the integration of DSS with emerging technologies like the Internet of Things (IoT) and artificial intelligence. This integration can lead to more advanced predictive models and simulation scenarios, driving better decisions based on real-time data. Additionally, further studies could explore how to optimize user training and engagement with DSS, as well as strategies for improving user acceptance across diverse farming contexts.
"DSS can fundamentally change the way farmers interact with data, moving towards a more informed approach in every decision made."
Ultimately, the exploration of Decision Support Systems in agriculture serves as a roadmap for future innovations. By recognizing their current limitations and potential growth areas, stakeholders can work towards fully realizing the benefits these systems promise.