Innovative Experimental Design in Agriculture
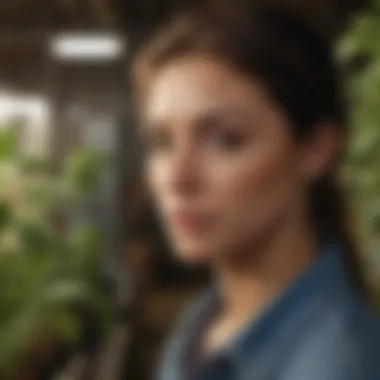
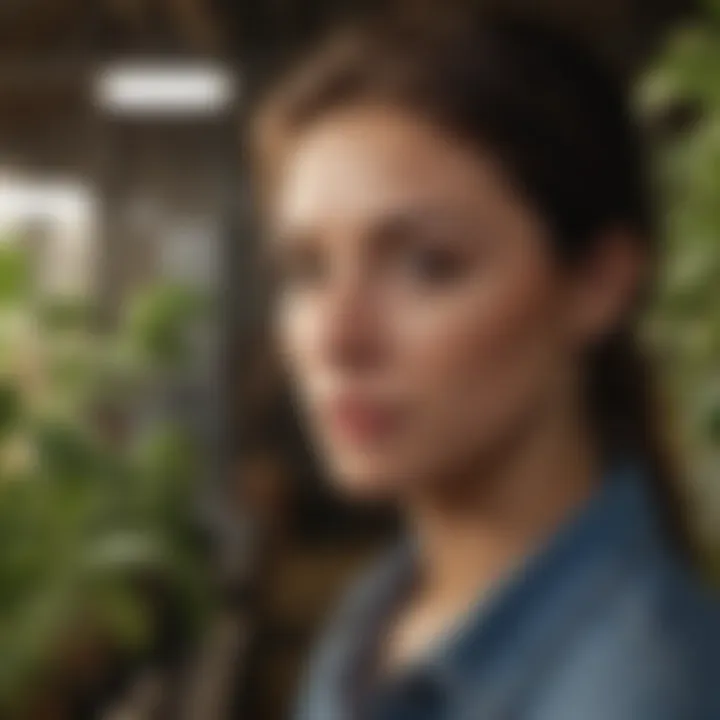
Intro
Agriculture is a foundational industry that shapes economies and sustains populations globally. As the demand for food increases due to rising populations and changing dietary preferences, the sector faces continuous pressure to boost productivity while maintaining sustainability. Here, the importance of experimental design and analysis comes into play. It forms the backbone of effective research and implementation in agriculture, influencing everything from crop management practices to livestock health. This article examines the methodologies that empower farmers and agricultural researchers to make informed decisions.
Overview of the Topic
Definition and Importance
Experimental design refers to the systematic planning of research studies aimed at answering specific questions. In agriculture, this means constructing experiments that generate valid and reliable data about various factors influencing crop yields, pest control, and soil health. The importance of this discipline cannot be overstated. It helps in identifying cause-and-effect relationships, leading to optimized practices and greater efficiency in resource use. Additionally, robust experimental designs can improve sustainability and increase resilience against climate change.
Current Trends
In recent years, there has been an increasing emphasis on integrating technology into experimental design. Precision agriculture tools, such as drones and IoT devices, allow for the collection of high-resolution data. This data can be analyzed using advanced statistical methods, providing deeper insights into experimental outcomes. Furthermore, the collaboration between agronomists and data scientists is fostering innovations in methodologies, enhancing the reliability of results.
Key Techniques and Practices
Step-by-Step Guide
To begin, designing an experiment in agriculture typically follows several key steps:
- Identify the Research Question: A clear and concise question forms the basis of any good experiment.
- Define Variables: Distinguish between independent variables (manipulated) and dependent variables (measured).
- Choose an Experimental Design: Options include randomized controlled trials, block designs, and factorial designs, each with its advantages depending on the research question.
- Randomization: Assign treatments randomly to reduce bias and ensure the results are generalizable.
- Data Collection: Collect data meticulously, monitoring for consistency and accuracy throughout the experiment.
- Data Analysis: Use appropriate statistical tools to analyze the data and determine if the results are statistically significant.
Tools and Equipment Needed
A range of tools and equipment are essential for conducting experimental agriculture:
- Statistical Software: Programs such as R and SPSS are crucial for data analysis.
- Measurement Tools: Soil moisture sensors, yield monitors, and weather stations facilitate precise data collection.
- Field Experimentation Supplies: Items like plots, markers, and sampling equipment are necessary for on-ground experiments.
Challenges and Solutions
Common Obstacles
While the benefits of experimental design in agriculture are significant, challenges still remain. Some of the common obstacles faced include:
- Limited access to technology in certain regions.
- Difficulty in controlling environmental variables, which can introduce noise in data.
- Time constraints related to seasonal variations and planting schedules.
Innovative Solutions
Innovative solutions are being developed to address these challenges:
- Collaborative Efforts: Partnerships between farmers, researchers, and tech companies can improve access to essential tools and software.
- Adaptive Design: Employing adaptive trial designs allows for modifications during the experiment to address unforeseen challenges.
- Training and Workshops: Educating farmers on experimental design methods can lead to better implementation and innovative thinking in traditional farming practices.
"By embracing experimental design, farmers can make data-driven decisions that significantly enhance productivity and sustainability in agriculture."
Foreword to Experimental Design in Agriculture
Experimental design stands as a cornerstone in agricultural research, guiding researchers in evaluating hypotheses and improving farming practices. The systematic approach provided by experimental design helps in organizing and interpreting data effectively, which can lead to critical advancements within agriculture. As pressures from climate change, population growth, and declining resources mount, well-structured designs in experimentation become necessary for ensuring sustainable and productive agricultural practices.
This section will delve into two significant aspects of experimental design: its definition and its importance in agricultural research.
Defining Experimental Design
Experimental design refers to the process of planning an experiment effectively to test a hypothesis. It involves deciding how to collect, control, and analyze data. A well-defined experimental design enhances the reliability of results by reducing biases and ensuring that the outcomes can be attributed to the factors being tested. In agriculture, this can mean the difference between understanding crop yield variations and merely documenting them.
A few critical components of experimental design include:
- Hypothesis formulation: Clearly stating the hypothesis to be tested.
- Selection of variables: Identifying independent and dependent variables, along with control factors.
- Choice of design: Choosing among different experimental designs such as randomized block or factorial designs that best fit the research question.
- Data collection methods: Determining how data will be collected and measured.
These elements work together to guide the researcher towards obtaining valid and insightful results, which can drive further innovations in agricultural practices.
Importance in Agricultural Research
Agricultural research increasingly relies on experimental design to produce robust data that leads to informed decision-making.
The relevance of well-planned experiments can be seen across several key areas:
- Improving crop yield: Through systematic testing, researchers can identify the most effective farming methods, fertilizer application, and pest control strategies.
- Resource management: Experiments can help assess the efficiency of water and soil use, ensuring these vital resources are used optimally.
- Adapting to climate change: Experimental design allows researchers to simulate different climate scenarios, leading to the development of resilient crop varieties.
- Policy formulation: Robust experimental data can inform policies aimed at sustainable agricultural practices, benefiting both the environment and the economy.
"A solid experimental design provides a framework for gathering valid, actionable data that addresses the pressing challenges in agriculture today."
In summary, the significance of experimental design in agriculture cannot be overstated. By establishing a clear framework for testing and analyzing various agricultural practices, researchers can contribute significantly to the ongoing evolution of the agricultural industry, ensuring that it meets the demands of future generations.
Foundations of Experimental Design
The foundations of experimental design serve as the framework for conducting credible agricultural research. A solid understanding of these principles is vital for designing experiments that yield meaningful results. This section emphasizes key terminology and essential principles of experimental design that guide researchers in systematic investigation. Knowing these elements allows research to address specific agricultural problems effectively and to draw valid conclusions.
Key Terminology
Understanding the vocabulary used in experimental design is crucial. Key terms include:
- Independent Variable: This is the factor that researchers manipulate to observe its effect.
- Dependent Variable: The outcome researchers measure to determine the effect of changing the independent variable.
- Control: A standard, unchanged condition used for comparison.
- Treatment: The specific conditions or interventions being tested.
Familiarizing oneself with these terms is essential for both researchers and practitioners in agriculture, as it facilitates clear communication and understanding of experimental processes.
Principles of Experimental Design
Principles of experimental design guide researchers in creating robust experiments. Key principles include:
Randomization
Randomization involves assigning subjects or experimental units to different treatment groups using random methods. This minimizes bias in experiment outcomes, allowing for a more accurate analysis. The key characteristic of randomization is its ability to create groups that are statistically comparable. It is beneficial in agricultural studies as it helps to mitigate the effects of confounding variables, which may otherwise skew results.
A unique feature of randomization is its straightforward implementation. While its advantage is clarity and simplicity in design, a potential disadvantage might be logistical challenges in larger scales of study.
Replication

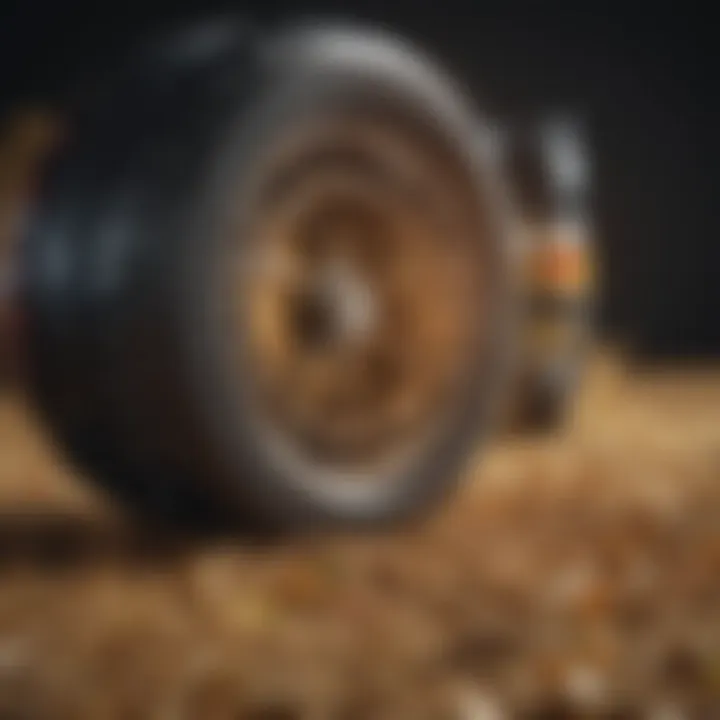
Replication refers to repeating an experiment or treatment to confirm findings. This principle is crucial because it enhances the reliability of results. The key characteristic of replication is that it reduces the impact of random errors, increasing confidence in the outcomes. Replication is beneficial, especially in agriculture, where environmental variability can significantly influence results.
The unique feature of replication is that it provides a way to test whether observed effects are consistent. However, one drawback can be the need for additional resources, such as time and funding, to conduct multiple trials.
Blocking
Blocking is a technique used to account for variability among experimental units. In this approach, similar subjects are grouped into blocks, and treatments are randomly assigned within these blocks. The key characteristic of blocking is its ability to increase the precision of the experiment. It is particularly beneficial in agricultural contexts, where field variability may influence results.
A unique feature of blocking is that it allows researchers to control for extraneous variables that may affect the dependent variable. This can lead to more accurate results. However, creating blocks can complicate design and may require significant planning.
Understanding the foundations of experimental design equips agricultural practitioners to conduct effective research, ultimately improving farming practices.
Types of Experimental Designs
Understanding the different types of experimental designs is crucial for anyone involved in agricultural research or practice. These designs serve as a framework for structuring experiments, allowing for effective data collection and analysis. Each design type offers specific benefits and considerations, shaping how results are interpreted. The choice of design directly affects the validity and reliability of findings, making it essential to comprehend which design fits best based on the goals of the research.
Completely Randomized Design
The Completely Randomized Design (CRD) is a foundational experimental design that emphasizes the random allocation of treatments to experimental units. This method ensures that every unit has an equal chance of receiving any treatment, reducing bias.
Advantages of CRD include:
- Simplicity: The design is straightforward to implement, making it accessible for studies with fewer resources or complexity.
- Flexibility: It accommodates various treatments and experimental conditions, allowing researchers to explore numerous variables.
However, this design can be limiting in scenarios where inherent variability among experimental units exists. CRD may not address confounding factors effectively, which could affect the experiment's outcomes.
Randomized Block Design
Randomized Block Design (RBD) improves upon CRD by reducing the variability among experimental units. In this design, units are grouped into blocks based on a specific characteristic, such as soil type or geographical area. Each treatment is randomly assigned within these blocks.
Key benefits of RBD include:
- Control of Variability: Blocking helps to control variance caused by nuisance factors, providing more accurate estimates of treatment effects.
- Improved Precision: The ability to account for variability enhances the precision of the treatment effect estimates.
RBD is especially useful in agricultural studies where the environment can affect results significantly. The design allows for a more focused examination of how treatments perform across controlled conditions.
Factorial Design
Factorial Design takes a comprehensive approach by examining the effects of multiple factors simultaneously. Each factor can have different levels, allowing researchers to observe interactions between them. This design is particularly effective for complex agricultural experiments where multiple variables may influence outcomes.
The essential aspects of Factorial Design include:
- Interactivity Assessment: The ability to study interactions between factors provides deeper insights into how they influence each other.
- Efficiency: The design allows for a more efficient use of experimental resources by exploring several variables at once.
Despite its advantages, experimentation through Factorial Design can become intricate as the number of factors increases, making analysis and interpretation of results more challenging.
Split-Plot Design
Split-Plot Design is used in scenarios where it is difficult to manage randomization at a small scale. This design involves two levels of experimental units. The main plots receive one treatment, while subplots within those main plots receive different treatments.
Noteworthy points include:
- Practicality in Field Conditions: Split-Plot Design is often more practical in large agricultural settings where the complete randomization is unfeasible.
- Consideration of Larger Variability Sources: This design allows researchers to investigate both main plot effects and subplot effects, thus accommodating variability across different scales.
However, analysis can be complex due to different levels of replication and randomization.
"Choosing the appropriate experimental design is pivotal in ensuring that agricultural research translates into effective practices in the field."
Each of these designs carries distinct strengths that make them suitable for varied research objectives in agriculture. Selecting the right design requires a careful consideration of the research question, available resources, and the nature of the experimental conditions.
Choosing the Right Design
Choosing the right experimental design is a critical step in agricultural research. An appropriate design not only streamlines the process but also enhances the validity of the results. Each experimental design has distinct strengths and weaknesses, and understanding these can lead to better decision-making. This section focuses on two specific elements: assessing research objectives and considering available resources.
Assessing Research Objectives
Understanding the specific aims of a research project is vital for choosing an appropriate experimental design. Agriultural research often seeks to answer complex questions related to crop yields, pest resistance, or soil health. When researchers establish clear objectives, it becomes easier to select a design that aligns with these goals. For example, if the goal is to evaluate the performance of different fertilizers, a Randomized Block Design may be used to account for variability in soil conditions across different plots. This tailored approach enhances the reliability of findings.
Additionally, researchers should consider if they are testing a single variable or multiple factors simultaneously. Factorial designs work well in scenarios where the interaction between different variables, such as irrigation levels and nutrient types, might be significant. Conversely, simplistic objectives can often be addressed satisfactorily with a basic Completely Randomized Design.
In essence, assessing research objectives is about asking the right questions and identifying the best path to finding the answers.
Considering Available Resources
Every research project operates within constraints, and available resources can significantly influence the choice of experimental design. Resources include time, funding, space, and access to technology. For example, if a researcher has limited funding, implementing a complex design with multiple treatment levels and numerous replications may not be feasible.
Instead, options such as Split-Plot Design could allow for the examination of both whole plots and sub-plots more economically. Itโs essential to match the chosen design with the available tools and personnel. The implementation of big data techniques might require more sophisticated data collection and analysis resources than simpler designs would demand.
Moreover, practical constraints should not be overlooked. Locations, seasonal variations, and equipment availability can impact the success of an experimental design. Recognizing these limitations can guide researchers in their choices, ensuring that the selected design is not only scientifically valid but also practically achievable.
"Effective experimental design is not just about applying the right statistical techniques; itโs about aligning them with real-world constraints and objectives."
In summary, careful consideration of both research objectives and available resources is vital when choosing an experimental design. This alignment ensures that the resulting study can yield meaningful and actionable insights. When executed properly, the right design can enhance the relevance and applicability of agricultural research findings.
Data Collection Techniques in Agricultural Experiments
Data collection is a fundamental aspect of experimental design in agriculture. It serves as the backbone for evaluating efficacy, understanding dynamics within ecosystems, and shaping future agricultural practices. The methods used for data collection can greatly influence the outcomes of research and provide vital information to agricultural practitioners. Effective data collection techniques lead to more informed decision-making and can make the difference between success and failure in farming endeavors.
Moreover, the right techniques can enhance the reliability of the results. Thus, understanding both quantitative and qualitative methods is essential. It is important for farmers and researchers to consider the objectives of their studies when selecting their approaches. Incorporating diverse data sources can provide a more rounded understanding of agricultural phenomena.
Quantitative Methods
Quantitative methods involve the use of structured tools that produce numerical data. These methods allow researchers to analyze and interpret information statistically. Systematic surveys, field trials, and experiments fall under this category. They deliver measurable outcomes, which can be subjected to statistical testing.
Some common quantitative data collection techniques include:
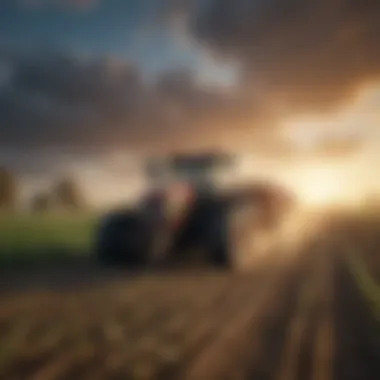
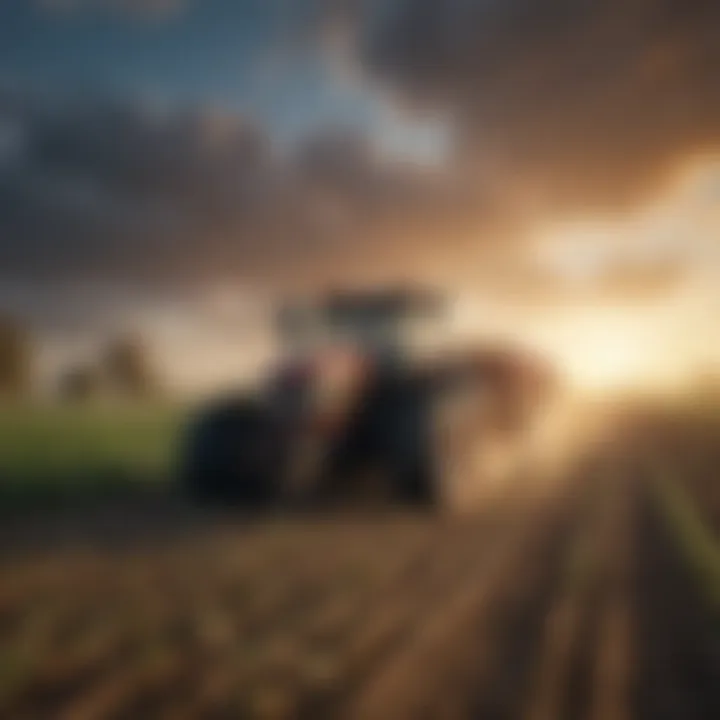
- Surveys and Questionnaires: These tools gather information on various variables affecting agriculture, such as soil health, crop yield, and pest prevalence. They help gather large amounts of data swiftly.
- Controlled Experiments: Experimental designs wherein factors are manipulated to see their effect on an outcome. For example, a study might alter irrigation levels to assess their impact on crop yield.
- Remote Sensing: This technology uses satellite or aerial imagery to gather data on crop health, soil conditions, and environmental variables. It provides insights over large areas quickly.
Utilizing these quantitative methods not only enables researchers to gain precise insights but also facilitates comparisons over time and across different geographical areas. By gathering and analyzing solid numerical data, farmers can make evidence-based decisions that enhance productivity.
Qualitative Methods
On the other hand, qualitative methods focus on understanding the reasons and motivations behind various agricultural practices. They are more subjective and often involve observation, interviews, and content analysis. These techniques provide context to the numerical data collected through quantitative methods.
Key qualitative data collection techniques include:
- Interviews: Engaging with farmers, agronomists, and local experts can reveal insights that numbers alone cannot provide. Personal narratives often highlight challenges faced in the field.
- Focus Groups: Group discussions can bring out collective experiences and opinions. This method encourages dialogue on specific issues, such as sustainable practices or innovation adoption.
- Field Observations: Observing farming practices and environmental interactions firsthand can uncover underlying issues that might not be part of structured surveys.
Qualitative methods can enhance the overall understanding of agricultural systems. They capture the intricacies of stakeholders' interactions with their environment. Using these methods, farmers can adapt their practices based on real-world experiences and observations.
Statistical Analysis in Agricultural Research
Statistical analysis serves as the backbone for sound decision-making in agricultural research. It enables researchers and practitioners to derive meaningful insights from data, thus improving the efficiency and sustainability of farming practices. By utilizing statistical methods, agriculturalists can draw reliable conclusions from their experiments, assess trends, and ultimately enhance productivity.
The benefits of statistical analysis in agriculture include:
- Data Interpretation: Agricultural data is often complex. Statistical tools help in simplifying this data, allowing for clearer insights.
- Hypothesis Testing: Statistical methods allow for rigorous testing of hypotheses, aiding in validating new techniques or interventions.
- Predictive Modeling: Through statistical techniques, farmers can predict yields, pest outbreaks, and other critical factors influencing agricultural success.
When engaging in statistical analysis, there are several considerations that must be taken into account. These include:
- Choosing Appropriate Techniques: Different types of data require different statistical methods. Selecting the right technique is crucial.
- Understanding Assumptions: Each statistical method has its own assumptions. It is important to determine if data meets these conditions.
- Importance of Sample Size: A sufficiently large sample size ensures that the statistical results are credible and generalizable.
Prologue to Statistical Methods
Statistical methods provide the framework for analyzing agricultural data. They encompass a range of techniques designed to collect, process, and interpret information.
In agriculture, some common statistical methods include:
- Descriptive Statistics: Summarizes data measurements and patterns, offering insights into mean, median, and standard deviation.
- Inferential Statistics: Helps make predictions or generalizations about a population based on sample data.
- Multivariate Analysis: Examines the relationship between multiple variables, which is crucial for understanding complex interactions in agriculture.
With the evolving landscape of agricultural science, reliance on these methods is growing. Utilizing suitable statistical approaches enhances the validity of results and supports better decision-making.
ANOVA in Experimental Design
Analysis of Variance, commonly known as ANOVA, is a powerful statistical method used to compare the means of three or more groups. This technique is particularly beneficial in agricultural research for comparing various treatments or conditions.
Key advantages of using ANOVA in experiments include:
- Efficiency: ANOVA can handle multiple groups simultaneously, reducing time and resource expenditure compared to multiple t-tests.
- Insight into Variability: It not only assesses the differences in means but also provides insight into the sources of variability in the data.
When performing ANOVA, it is essential to check for the following conditions:
- Normality: The data should be approximately normally distributed.
- Homogeneity of Variances: Groups should have similar variances to validate the results of the analysis.
- Independence: Observations should be independent of each other.
Overall, ANOVA equips agricultural scientists with a robust tool to assess the effectiveness of different treatments systematically.
Regression Analysis Applications
Regression analysis is another fundamental statistical technique that plays a crucial role in agricultural research. It is used for modeling the relationship between a dependent variable and one or more independent variables.
This method offers several applications in agriculture:
- Yield Prediction: By using variables such as weather conditions, soil types, and crop management practices, regression models can effectively predict crop yields.
- Resource Allocation: Regression analysis assists farmers in understanding how adjustments in inputs influence outputs, leading to optimized resource allocation.
- Trend Analysis: Assessing long-term trends in crop production helps in strategic planning and adaptation to climate changes.
Like ANOVA, regression analysis also requires careful consideration of assumptions, such as linearity and homoscedasticity. Ensuring these assumptions hold true enhances the credibility of the findings.
"Statistical analysis is not just a tool; it is a framework for understanding and improving agricultural practices."
Common Pitfalls in Experimental Design
In agricultural experimental design, recognizing the potential pitfalls is crucial. These mistakes can undermine research efforts, leading to invalid conclusions and ineffective practices. Addressing these common mistakes will help ensure that studies yield reliable results, which can ultimately enhance agricultural productivity and sustainability.
Design Flaws
Design flaws are fundamental errors in the experimental setup that can compromise the integrity of the results. A well-structured design is essential to control for variables that might affect outcomes. Common flaws include inadequate randomization, which can bias the results by not equally representing all populations. Additionally, poor selection of controls can distort the comparison between treatment groups, leading to misleading interpretations.
Another significant flaw is a poorly defined sample size. Insufficient replication can lead to high variability which hides the actual treatment effects. Conversely, overly complex designs may confuse data interpretation. Striking a balance between simplicity and comprehensiveness in design is vital. It helps assure the methods used are appropriate for the experimental objectives.
In short, addressing design flaws is critical for producing reliable, reproducible results in agricultural experiments.
Data Mismanagement
Data mismanagement poses a serious threat to the validity of agricultural research. This can involve many issues such as incorrect data entry, failure to properly store data or not following appropriate protocols during data collection. For instance, if field measurements are taken inconsistently, the resulting data set may not accurately reflect the true findings of the experiment.
Furthermore, inadequate data analysis can misrepresent results. Researchers must understand the statistical methods relevant to their data types. Improper use of methods like ANOVA or regression can lead to wrong conclusions about relationships or effects.
The significance of maintaining rigorous data management practices cannot be overstated. Keeping organized records, validating data entry, and applying appropriate analytical methods ensure that the results of agricultural experiments are credible and valuable.
"Effective experimental design is just as crucial as the execution of the experiment itself. Both elements must be seamless to produce robust conclusions."
In summary, being aware of common pitfalls such as design flaws and data mismanagement is essential for horticulturists and agricultural researchers. By implementing best practices, practitioners can enhance the reliability and applicability of their experimental findings.
Emerging Technologies in Data Analysis
Emerging technologies are redefining how data is analyzed in agriculture. These new methods offer precise tools that enhance accuracy and efficiency in agricultural practices. Big Data and machine learning are at the forefront of these innovations, providing valuable insights that can lead to more informed decision-making and better outcomes in farming.
Role of Big Data
Big Data refers to large volumes of data that cannot be processed effectively using traditional data processing software. In agriculture, Big Data plays a critical role in gathering information from various sources, including sensors, satellites, and farming equipment. Here are key points related to its impact:
- Precision Farming: Farmers can analyze weather patterns, soil conditions, and crop health in real-time. This leads to better decision-making and optimized inputs, enhancing yield and reducing waste.
- Market Insights: Big Data helps in predicting market trends, allowing farmers to align production with demand, ultimately maximizing profits.
- Risk Management: By analyzing vast datasets, farmers can identify potential risks, such as pest outbreaks or drought, and take proactive measures.
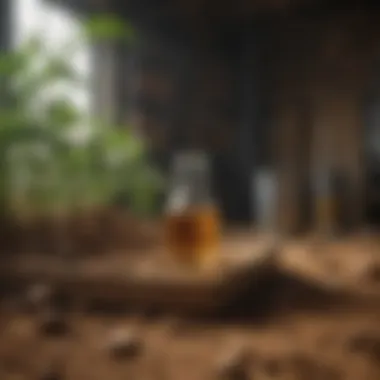
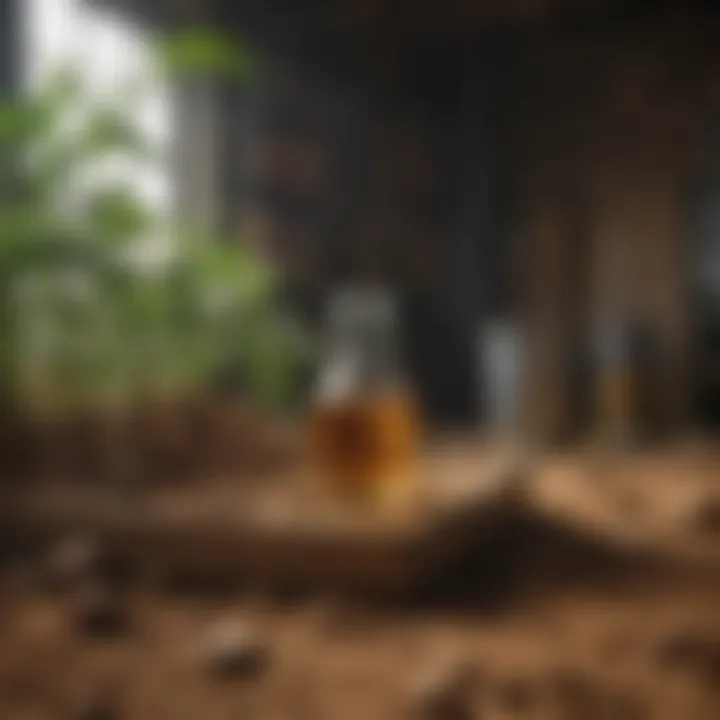
"The integration of Big Data technologies fosters a data-driven approach in agricultural practices, leading to sustainability and higher productivity."
Machine Learning Applications
Machine learning is a subset of artificial intelligence that enables computer systems to learn and adapt from experience without explicit programming. Its application in agriculture is varied and powerful:
- Predictive Analytics: Machine learning algorithms can predict crop yields based on historical data and variables affecting growth. This aids farmers in planning and resource allocation.
- Disease Detection: Machine learning models are deployed to analyze images of crops. They can identify diseases and nutrient deficiencies early, helping in timely interventions.
- Automation: Automated systems using machine learning can manage irrigation schedules and optimize fertilizer applications, reducing labor and resource expenditure.
The future of agricultural data analysis lies in these emerging technologies. Their ability to provide tailored insights and enhance operational efficiency is crucial in driving agricultural advancements. As these technologies continue to evolve, they offer substantial opportunities for farmers and stakeholders in the agricultural sector.
Case Studies in Agricultural Experimental Design
Case studies serve as vital examples in agricultural experimental design. They provide practical insights that enrich theoretical knowledge with real-world applications. By analyzing specific case studies, researchers and practitioners can evaluate methodologies employed in diverse settings. This analysis often highlights their effectiveness in addressing various agricultural challenges. Moreover, these cases illuminate the practical implications of experimental design principles, broadening the understanding of how structured experiments can lead to better farming practices.
Successful Applications
Successful applications in agricultural experimental design showcase innovative strategies to improve crop yields and sustainability. For instance, a study conducted on rice production utilized a randomized complete block design to evaluate different fertilization techniques. Researchers observed that the application of organic fertilizers significantly enhanced yield compared to synthetic options. This application not only demonstrates the benefits of experimental design but also encourages the adoption of environmentally friendly practices among farmers.
Another example is the use of factorial design in a vegetable crop trial. The researchers tested multiple varieties of tomatoes, adjusting the irrigation levels alongside different soil types. The results provided insightful correlations between variety performance, irrigation, and soil conditions. Such case studies demonstrate how a structured design leads to actionable insights, effectively guiding farmers in optimizing their production strategies.
Lessons Learned
Through various agricultural case studies, several lessons emerge that can shape future research and practice in the field. First, the importance of local context cannot be overstated. Experiments designed in one region may not yield the same results in another due to differing environmental conditions and local farming practices. This underscores the necessity of contextualizing research findings within specific geographical and cultural frameworks.
Second, effective data collection and management are critical for deriving meaningful conclusions. A case study in precision agriculture highlighted how improper data handling led to flawed results, hindering the ability to scale findings. Such instances stress the need for thorough training in data management for agricultural researchers.
Finally, collaboration among stakeholders emerges as a recurrent theme. Successful applications often involve partnerships between researchers, local farmers, and agricultural extension services. This collaborative approach fosters knowledge-sharing and facilitates the implementation of best practices. By learning from these lessons, agricultural practitioners can refine their experimental designs, leading to more effective outcomes in sustainable practices.
"Using case studies enables us to learn from our successes and failures, shaping a more informed approach to experimental design in agriculture."
In summary, case studies in agricultural experimental design are crucial. They provide valuable insights into successful applications and lessons that can inspire future research and practices. By examining real-world examples, the agricultural community can enhance their methodologies, ultimately driving progress in farming.
Implications for Sustainable Agriculture
Sustainable agriculture has become crucial in modern farming practices. Experimental design and analysis play a key role in achieving sustainability. This is because they enable researchers and farmers to create structured methods for testing agricultural hypotheses. This structured approach leads to improved decision-making, efficient resource management, and enhanced productivity.
Integrating Design with Ecological Practices
Integrating experimental design with ecological practices is paramount. This integration allows farmers to assess the interactions between various agricultural inputs and their effects on the environment. For example, using randomized block designs can help determine how different crop varieties adapt to local ecosystems. By analyzing data, farmers can identify best practices that enhance biodiversity while ensuring soil health. This not only promotes crop yields but also minimizes negative environmental impacts.
Benefits include:
- Enhanced soil quality: Experimental methods such as crop rotation and the use of cover crops help maintain soil structure and fertility.
- Diversified crops: Varied species minimize pests and diseases naturally.
- Water conservation: Strategies like drip irrigation can be evaluated and optimized through careful experimental setups.
Enhancing Yield through Strategic Planning
Strategic planning is essential for increasing agricultural yields. Employing statistical analysis in experimental design allows for the identification of optimal planting times, plant densities, and nutrient applications. For example, factorial design can optimize multi-variable experiments to understand the interactions between variables like water availability and fertilizer types.
Farmers can utilize insights from these analyses to make informed decisions. They can allocate resources efficiently, resulting in higher yields with less waste. Furthermore, this approach supports long-term sustainability goals by promoting practices that allow continued productivity without depleting natural resources.
Key strategies include:
- Monitoring growth patterns: This involves keeping track of the performance of different crop types across various conditions.
- Adjusting inputs accordingly: Farmers can modify their practices based on real-time data to enhance productivity.
- Long-term evaluations: Implementing experiments that assess the outcomes of practices over multiple growing seasons allows for better strategic planning.
To summarize, integrating experimental design with sustainable practices enhances overall productivity while minimizing environmental footprints. The ongoing evaluation and strategic modifications lead to better resource utilization, yielding benefits not only for farmers but for the ecosystem as a whole.
Future Directions in Experimental Design
The field of experimental design in agriculture is rapidly evolving. As new challenges arise, it becomes essential to embrace innovative methodologies that enhance research effectiveness. Understanding future directions equips agricultural researchers and practitioners with the necessary tools to address pressing issues in food security, sustainability, and productivity.
Innovative Approaches Emerging
Recent advancements in technology have catalyzed innovative approaches in experimental design. Notably, the integration of precision agriculture plays a significant role. This approach uses technology to monitor field variability in crops and to optimize their management. These systems utilize data from multiple sources, including weather forecasts, soil moisture sensors, and crop health imaging. This can help farmers make timely decisions that improve yields.
Another noteworthy direction is the rise of adaptive experimental designs. Unlike traditional designs that fix parameters before experimentation begins, adaptive designs allow modifications as data is collected. This is particularly useful in long-term studies, where initial assumptions might change as new insights are gained. Such flexibility can lead to better resource allocation and improved outcomes.
Finally, participatory approaches are gaining attention. Involving farmers in the design and execution of experiments not only fosters local knowledge but also enhances the relevance of findings. Direct engagement can lead to practical solutions that are more readily adopted in communities, bridging the gap between research and application.
The Role of Remote Sensing
Remote sensing is revolutionizing agricultural research and experimental design. It refers to the use of satellite or aerial imagery to collect data about agricultural areas without direct contact. This technology provides high-resolution data on crop health, soil conditions, and environmental factors.
One major advantage of remote sensing is the ability to cover large areas efficiently. It enables researchers to observe widespread patterns that would be impossible to evaluate with ground-based methods alone. This can inform experimental designs by highlighting zones of interest or variation within a study area. For instance, understanding microclimates can enhance the precision of crop trials.
Moreover, remote sensing facilitates real-time monitoring. By integrating this technology into experimental setups, researchers can immediately address variations or unexpected results. This immediacy can be vital for quick adjustments in management practices or experimental protocols.
Incorporating remote sensing data into traditional experimental design frameworks can enhance the understanding of environmental interactions and crop responses. As this technology continues to advance, its integration into agricultural experimental designs will likely become a norm, paving the way for more informed decisions and improved agricultural practices.
"The future of agricultural design lies in its ability to adapt and incorporate emerging technologies. Staying ahead will ensure better trial results and sustainable practices."
Culmination
The conclusion draws together the various strands of experimental design and analysis discussed throughout the article. It highlights the necessity of these methodologies in modern agriculture, emphasizing their role in improving productivity and sustainability. Effective experimental design is paramount for testing hypotheses, optimizing farming practices, and ultimately enhancing crop yields. This structured approach not only guides researchers but also informs farmers in making evidence-based decisions that affect their daily operations.
Another critical benefit of sound experimental design is its contribution to resource efficiency. In an era where agricultural outputs must increase without proportionately increasing environmental impacts, such design frameworks help identify best practices tailored to specific conditions. As agricultural challenges evolve, an adaptable experimental design can provide insights that lead to innovative solutions.
Moreover, the integration of new technologies into experimental design, like big data and machine learning, adds considerable value. These advancements offer nuanced insights into agricultural systems and improve the accuracy of predictions regarding best practices. Therefore, practitioners who embrace these approaches position themselves at the forefront of a rapidly advancing field, capable of driving future innovations in agricultural methods.
In summary, the importance of rigorous experimental design cannot be overstated. It serves as the keystone for achieving sustainable agricultural advancements.
Summary of Key Insights
- Importance of Experimental Design: The article emphasizes that well-structured experimental design is essential for agriculture, allowing for the effective testing of hypotheses and improving methods.
- Types of Designs: Familiarity with various designs like Randomized Block and Split-Plot Designs provides agricultural researchers and practitioners with tools critical for their specific requirements.
- Statistical Tools: Understanding statistical methods, including ANOVA and regression analysis, empowers readers to analyze data correctly and derive actionable insights from their results.
- Emerging Technologies: The integration of big data and machine learning into agricultural research is highlighted as transformative for enhancing analysis and understanding of complex systems.
- Sustainable Practices: The discussion on how experimental design can align with ecological practices recognizes the need for sustainable approaches in food production.
Call to Action for Practitioners
Agricultural practitioners are encouraged to adopt the principles of experimental design in their work. Here are key steps to consider:
- Educate Yourself: Familiarize with concepts of experimental design and statistical analysis. Online courses and workshops can be highly beneficial.
- Implement Best Practices: Begin incorporating structured approaches while planning experiments for planting or livestock management.
- Leverage Technology: Utilize available technologies to collect and analyze data. Free tools can serve as a starting point before investing in advanced software.
- Collaborate: Work with professionals who have expertise in experimental design. Peer feedback can refine your methods and enhance your understanding.
- Share Findings: Contribute to knowledge by sharing outcomes from your experiments with the wider community, whether through platforms like Reddit or local agricultural forums.
By embracing these recommendations, farmers and agricultural scientists can make significant strides toward more effective and informed practices. This will not only benefit their operations, but also contribute to advancements in the entire agricultural sector.