Key Principles for Effective Agricultural Data Use
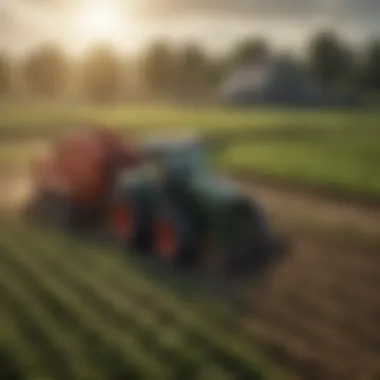
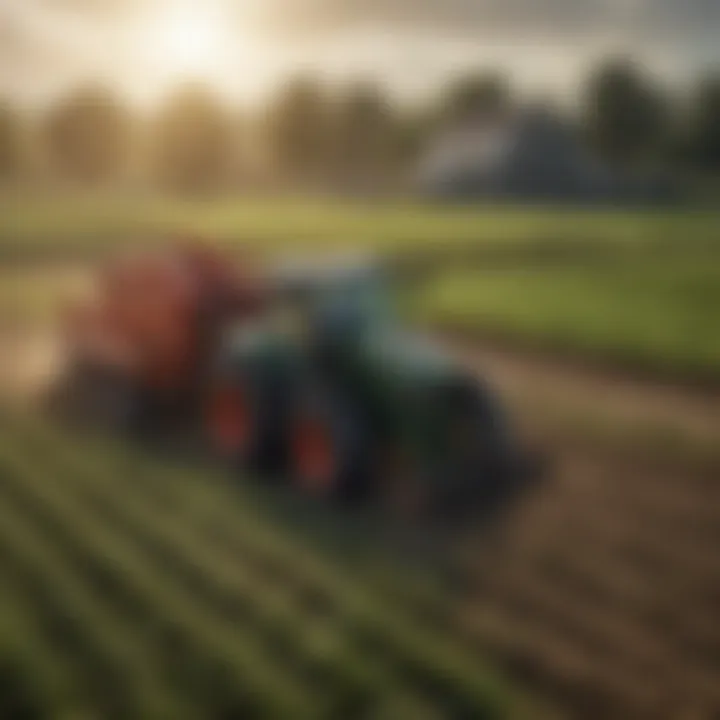
Overview of the Topic
Definition and Importance
In todayâs agricultural landscape, data has become a cornerstone of efficiency and productivity. When we talk about data guiding principles, we refer to a set of rules and practices that ensure the proper management and utilization of agricultural data. This encompasses everything from how data is collected to how it is shared and analyzed. With the rapid advances in technology, understanding these principles is paramount for farmers and agricultural professionals. Not only does reliable data help in decision-making, but it also fosters sustainable practices that can benefit the environment.
Consider a farm using soil health data to determine the best times to plant crops. If they rely on inaccurate or incomplete data, the outcome could lead to poor yields. Thus, the integrity of the data becomes a critical factor. In essence, sound data management aligns agricultural practices with broader goals of efficiency and sustainability.
Current Trends
The agricultural sector is experiencing significant shifts driven by technology. The rise of precision agriculture, for instance, has made it common to see farmers utilizing data analytics, remote sensing, and geographic information systems (GIS). These innovations help farmers optimize their resources, reducing waste and enhancing yield.
Some current trends include:
- Integration of IoT Devices: Smart sensors and devices gather real-time data to monitor various aspects of farming.
- Data Sharing Platforms: Collaborative platforms enable farmers to share insights, improving practices across the community.
- AI and Machine Learning: These technologies analyze vast datasets to predict outcomes and recommend best practices.
Such trends highlight a fundamental shift: agriculture is increasingly becoming a data-driven industry.
Key Techniques and Practices
Step-by-Step Guide
To effectively implement data guiding principles, farmers can follow these steps:
- Identify Key Metrics: Determine which data points are vital for your operations, such as crop yields, soil health, and weather patterns.
- Data Collection: Utilize tools like soil probes or weather stations to gather real-time data.
- Data Analysis: Use software solutions for analysisâthis can be anything from spreadsheets to advanced analytics programs.
- Decision Making: Apply the insights gained from your data to inform practices like crop rotation and pest control.
- Feedback Loop: Continuously monitor outcomes and refine your practices based on what the data tells you.
Tools and Equipment Needed
- Soil Sensors: Enable monitoring of soil moisture and nutrient levels.
- Drones: Can capture aerial imagery for crop surveillance and analysis.
- Data Management Software: Programs like FarmLogs or FieldView help in managing and visualizing data.
- GIS Tools: Essential for mapping and spatial analysis of agricultural data.
Challenges and Solutions
Common Obstacles
Despite the benefits, there are challenges in implementing data principles effectively. Some common obstacles include:
- Data Overload: With so much information available, it can be overwhelming to know what to focus on.
- Inconsistency: Variability in data sources can lead to conflicts in analysis and misinterpretation.
- Privacy Concerns: Sharing data can raise issues about the protection of sensitive information.
Innovative Solutions
Addressing these challenges requires strategic approaches:
- Streamlined Data Processes: Establish clear protocols for data collection and analysis. This can help reduce overwhelms and mark clear priority areas.
- Standardization: Use common metrics and formats when gathering data to ensure consistency.
- Robust Security Protocols: Ensure data privacy by implementing strong protective measures and transparency in data handling.
"The right data can transform not just farming techniques, but entire agricultural systems, fostering a future that is both productive and sustainable."
Finale
In closing, the guiding principles for data usage in agriculture offer a framework that can revolutionize the sector. By focusing on integrity, accessibility, and ethical considerations, practitioners can unlock the potential of data to enhance productivity while promoting sustainability. As agriculture continues to evolve, those who understand and embrace these principles will likely lead the way toward a more innovative agricultural future.
Intro to Data Guiding Principles
Understanding the cornerstones of data management is essential in agriculture, where the stakes are high, and precision matters. As farmers and agricultural professionals navigate an increasingly complex ecosystemâwhere every decision can affect yields, profit margins, and sustainabilityâthe guiding principles of data management come to the forefront.
The principles do not merely help in maintaining flow and organization; they underpin the very fabric of informed decision-making that drives productivity. By establishing a structured approach to data, one can transform raw figures into actionable insight that aligns with the goals of efficiency and resource management. In essence, these principles serve as a roadmap for practitioners seeking to leverage data with intention.
One must ponder, what makes data in agriculture different from other sectors? Itâs not just about collecting numbers; itâs about understanding the context behind them, from soil quality and weather patterns to market trends and pest occurrences. The knowledge embodies a language, and being fluent in it can significantly alter an agricultural operation's success.
Defining Data in Agriculture
Data in agriculture encompasses a wide array of information types. Broadly speaking, one can categorize it into two main types: quantitative and qualitative data. Quantitative data involves measurable elements such as crop yield, rainfall levels, and temperature readingsâthese numbers tell a story backed by hard facts. Conversely, qualitative data offers insights into the context, such as farmer experiences or soil health assessments that, although harder to quantify, provide depth to the understanding of agricultural practices.
Furthermore, technology has expanded the types available. From satellite imagery that tracks field changes to IoT devices monitoring crop conditions in real-time, farmers now have a treasure trove of data at their fingertips. This data landscape is constantly evolving, and understanding how to interact with these numerous data sources will determine how well one adapts in this complex environment.
Significance of Data Principles
The significance of data principles extends beyond compliance; they are crucial for fostering trust and transparency in agricultural practices. Here are several reasons why these principles hold substantial value:
- Accuracy: Data must be correct to be effective. When farmers understand the parameters that determine the quality of their data, they can make better choices.
- Consistency: Regularly collected data ensures that patterns can be recognized across different seasons, helping to establish benchmarks that set the standard for performance.
- Relevance: Principles guide users to focus on the data that truly matters for their specific agricultural conditions, eliminating noise that can obfuscate decision-making.
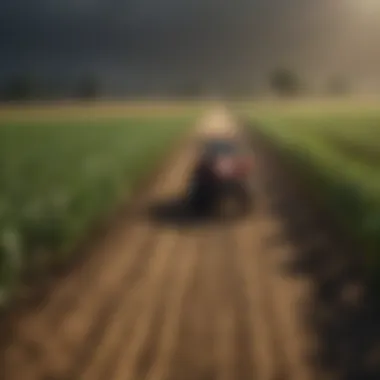
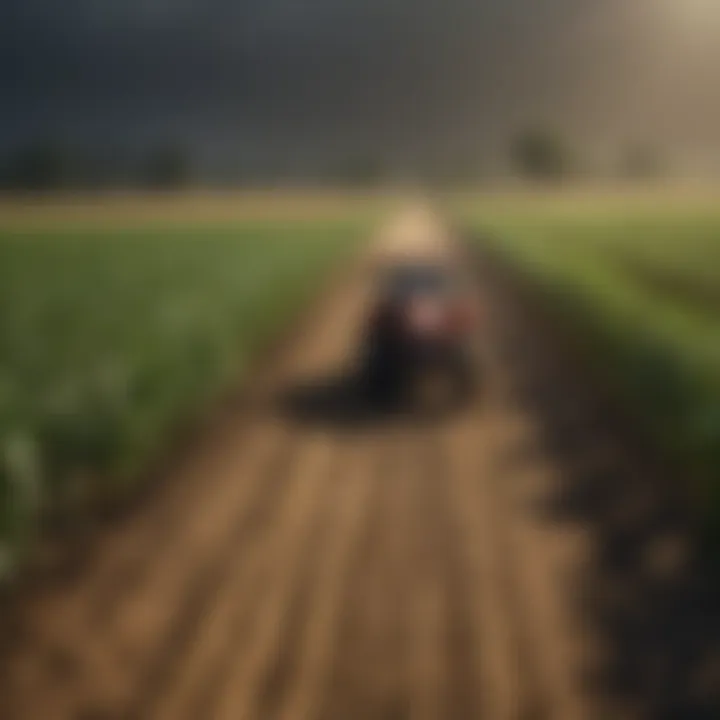
"Data without principles is like seed without soil; it lacks the foundation to grow and flourish."
In agricultural practices, applying these principles underscores an organizationâs commitment to quality and effectiveness. Moreover, these data principles encourage a culture where information is shared openly among stakeholdersâfrom farmhands to executivesâfostering a collaborative environment supportive of innovative practices.
Understanding Data Integrity
Data integrity is the bedrock of effective agricultural practices fueled by data. Without it, the entire edifice of decision-making crumbles. This concept generally refers to the accuracy and consistency of data over its lifecycle, and its significance in agriculture cannot be overstated. In a world where every seed planted or harvested relies heavily on data analysis, integrity becomes crucial. When data is reliable, farmers can accurately predict yields, manage resources more effectively, and respond to changing environmental conditions. Moreover, it ensures that the strategies employed are truly beneficial, instead of being based on faulty or skewed information.
Importance of Accurate Data Collection
When it comes to agriculture, accurate data collection isn't just a good practice; itâs a necessity. Farmers can catch a glimpse into their operations through the lens of collected data: crop health, soil quality, weather patterns. Each of these elements is a piece of a larger puzzle. If even one piece is flawed, the overall picture can be misleading.
- Understanding crop health: Regular monitoring through reliable sensors helps ensure that plants are given the right nutrients.
- Soil analysis: By collecting accurate data about soil composition, farmers can amend their plots more effectively, enhancing productivity.
- Weather stations: Real-time data about weather patterns can save crops from unexpected weather events.
Farmers often feel the pain when they invest in improper data collection methods. Missteps here can lead to wasted resources and missed opportunities.
Methods for Verifying Data Accuracy
Verification of data accuracy involves multiple strategies to ensure that the insights farmers depend upon are sound. It's a bit like checking your compass before setting out on a journeyâif the bearing is off, you'll end up wandering.
- Cross-Referencing: Comparing collected data against established benchmarks helps identify discrepancies. For instance, if soil pH values collected in one location diverge from a known database, it may prompt further investigation.
- Automated Systems: Utilizing precision agriculture tools can streamline data collection while ensuring accuracy through built-in calibration mechanisms.
- Regular Audits: Stepping back to regularly audit data collection processes ensures that any errors are caught early. The practice is akin to routine maintenance on equipment; it prevents costly failures down the line.
Each of these methods strengthens the bridge between data collection and actionable insights, allowing agricultural professionals to navigate their fields with clarity.
"Good data is one of agriculture's greatest assets; it lets farmers know what direction to move toward and how to maximize their efforts."
Nurturing data integrity cultivates a trusting environment where informed decisions replace guesswork, sowing seeds for future success.
Data Accessibility in Agricultural Practices
Accessing data in agriculture isn't just a nicety; it's a necessity. Data accessibility shapes the decisions that farmers and agronomists make, influencing everything from crop yields to pest management. When data is easily obtainable and interpretable, farmers can make informed decisions, optimize resources, and ultimately increase their productivity. In a world that is quickly shifting towards more sustainable practices, ensuring that everyone has access to relevant agricultural data is vital.
Barriers to Data Access
Despite its importance, several barriers still hinder access to agricultural data. These obstacles can be technological, regulatory, or even cultural.
- Technological constraints: In many regions, especially in developing countries, limited infrastructure hampers data access. Poor internet connectivity or lack of proper devices means that farmers may not be able to tap into valuable information.
- Economic factors: The costs associated with data services often deter farmers from pursuing them. Not all farmers have the financial backing to invest in technologies that facilitate data access, and this gap can severely limit opportunities for many.
- Data regulations: Complicated legal frameworks may restrict how data can be shared and used. Privacy concerns can make organizations wary of providing access, leading to a lack of shared knowledge that could benefit the entire agricultural community.
- Cultural hesitancies: In some communities, there may be a distrust of technology or fears that sharing data could somehow leave them vulnerable. This can foster resistance to adopting data-driven practices.
Strategies for Enhancing Accessibility
To improve data accessibility, itâs essential to implement targeted strategies that not only overcome existing barriers but also promote a culture of data sharing and usage.
- Investing in infrastructure: Governments and private entities need to work together to enhance internet connectivity in rural areas. By providing stable access to reliable technology, farmers can more easily gather and utilize data.
- Affordable data services: Encouraging companies to offer low-cost or subsidized data plans targeted at farmers can make a significant difference. When farmers see the direct benefits of data accessibility reflected in their yield and efficiency, they may be more inclined to adopt these services.
- Simplifying regulations: Streamlining data sharing laws can facilitate easier access to vital resources. Keeping the farmerâs perspective in mind will ensure that regulations support empowerment, rather than hinder it.
- Community workshops: Educating farmers about the benefits of data usage can help alleviate fears associated with technology. Workshops can demonstrate simple methods for collecting and using data effectively, transforming skepticism into enthusiasm.
"The more informed the farmer, the better the farmland can deliver."
Ethical Considerations in Data Use
In today's agricultural landscape, ethical considerations around data usage hold a significant place. Farming is no longer only about planting and harvesting; it intertwines with vast amounts of data. The ethical use of this data influences decisions that can impact not just profit margins but also future generations.
Fostering a culture that prioritizes ethics helps build trust, not only among farmers themselves but also with consumers. Understanding the sensitivity surrounding agricultural data, especially related to personal and operational aspects, is essential. Farmers must navigate the complexities of maintaining their integrity while maximizing productivity.
Data Privacy and Security
Data privacy and security stand out as pivotal elements in ensuring ethical data practices within agriculture. When farmers collect dataâfrom soil moisture levels to crop yieldsâthey gather sensitive information that could lead to significant competitive advantages if mishandled. This vulnerability calls for a robust approach to data management.
Farmers and agricultural producers should be aware of regulations like GDPR in Europe, which enforce strict guidelines on data privacy. These regulations prioritize individual rights to control their data and demand transparency. Failure to conform to these regulations can lead to hefty fines and loss of trust among customers.
To ensure data privacy, consider adopting these best practices:
- Limit Data Collecting: Collect only the essential data needed for operations, steering clear of extraneous information.
- Use Encryption: Protect sensitive data with encryption techniques, which make it unreadable to unauthorized individuals.
- Regular Audits: Conduct audits to assess data handling practices and identify any potential vulnerabilities.
"Transparency in data practices is not just a legal requirement but a moral obligation to clients and consumers, fostering trust and cooperation."
Cybersecurity Measures for Agricultural Data
In an ever-evolving digital landscape, the risk of cyber threats looms larger than ever. With the advent of high-tech farming solutions and data infrastructure, the agricultural sector is vulnerable to cyberattacks. Hackers might target systems storing proprietary farming data or even leverage information for ransomware attacks.
Implementing strong cybersecurity measures is non-negotiable for all agricultural enterprises. Key steps include:
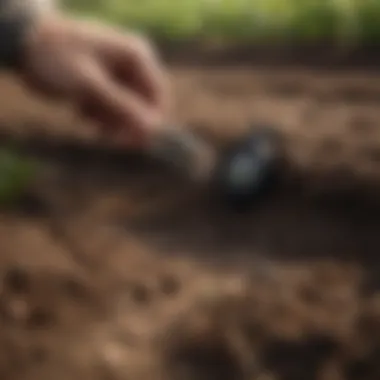
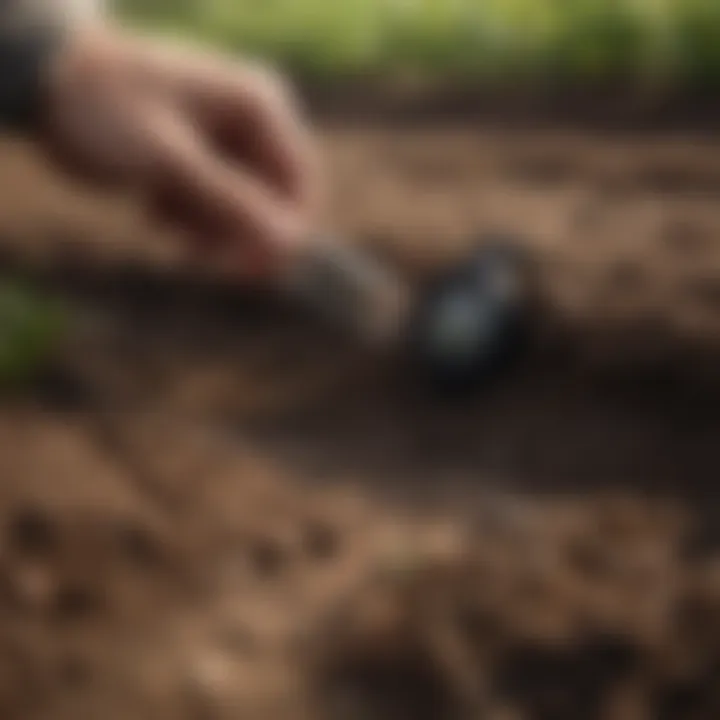
- Employee Training: Regularly educate staff on cybersecurity best practices, emphasizing the importance of recognizing phishing attempts.
- Backup Data: Regularly back up your data with secure cloud services or external hard drives. In the event of an incident, knowing your data is safeguarded is reassuring.
- Firewalls and Software Updates: Invest in reliable firewall protection and keep all software updated to mitigate vulnerabilities.
- Access Controls: Limit data access to authorized personnel only, reducing the risk of sensitive information being misused.
As agriculture increasingly incorporates technology such as IoT devices, taking proactive measures to strengthen security cannot be overstressed. The efforts employed towards ethical usage of data not only enhance operational efficiency but also safeguard the delicate fabric of trust in the relationship between data and stakeholders in agriculture.
Data-Driven Decision Making
In today's fast-paced agricultural landscape, data-driven decision making stands as a pivotal element that can vastly influence productivity and resource management. The modern farmer, increasingly faced with uncertainties stemming from climate variability, market fluctuations, and pest pressures, finds that leveraging data can unveil actionable insights that were previously out of reach. This approach allows for a more informed method of farming, steering clear of guesswork and allowing for adjustments based on empirical evidence. The integration of data into decision-making processes not only optimizes operations but also cultivates sustainability in an industry that often grapples with the strain of overexploitation.
Integrating Data into Agricultural Practices
Integrating data into agricultural practices is not merely about collecting numbers or statistics. It's about transforming raw information into potent strategies that drive the day-to-day operations of farming. Farmers equipped with tools that analyze weather patterns, soil health, and crop performance data can make more precise ag decisions. For instance, using sensors in fields can relay real-time information on soil moisture levels, helping farmers determine the optimal time for irrigation.
Further, data integration can extend to yield prediction models, which correlate historical yield results with current climatic conditions for more targeted crop management. When farmers can see these correlations clearly, they can plan for pests, adjust planting schedules, and even diversify their cropsâall based on what the data reveals.
Some specific practices for effective integration of data include:
- Utilizing Geographic Information Systems (GIS) to create detailed maps of fields.
- Regularly updating data tools and methodologies to keep pace with advancements in agriculture technology.
- Training staff and stakeholders to understand and use data effectively, ensuring that everyone is on the same page when it comes to decision-making.
By weaving data into the very fabric of agricultural practice, it becomes possible to foresee challenges before they arise, proactively adapt, and enhance the farm's efficiency.
Examples of Successful Data Applications
There are numerous real-world examples where data-driven decision making has markedly increased productivity in agriculture. One compelling case comes from precision agriculture. Farmers using data analytics in precision farming have been able to achieve grain yields previously thought unobtainable. By employing detailed mapping technologies to apply fertilizers in specific areas of the field rather than uniformly across, they have reduced waste and increased crop yields.
Another notable instance is the use of satellite imagery to monitor crop health. A farm in California successfully harnessed satellite data to identify areas of a vineyard that were under stress due to water inefficiencies. Following the insights garnered from the satellite analytics, they adjusted their irrigation techniques, resulting in a significant increase in grape yield and overall crop health.
Furthermore, companies like Trimble and Climate Corporation have developed software platforms for farmers that combine various data sourcesâfrom weather forecasts to soil metricsâthat drive everyday farming decisions. From this, farmers gain precise forecasts tailored to their specific fields, which assists in planning and deployment effectively.
"Data-driven decision making is not just a trend; it's a crucial pathway toward sustainable and efficient agricultural practices."
Utilizing Data for Crop Management
Utilizing data for crop management is not just a niche aspect of modern agriculture; it is the backbone that sustains growth and efficiency. As the agricultural landscape shifts due to climate change, market demands, and technological advancements, harnessing the power of data becomes ever more critical. The ability to track, analyze, and leverage data allows farmers to make informed decisions that lead to higher yields and better resource management.
Analyzing Crop Performance Data
Crop performance data can offer a wealth of insights into how plants are faring in various conditions. By collecting information on growth rates, disease incidence, and environmental factors, farmers can sharpen their focus on what works and what doesn't.
- Identifying Patterns: With the right tools, farmers can sift through vast amounts of data to spot trends over time. For example, if crop yields consistently drop during certain weather patterns, this could indicate a need for improved irrigation or soil management.
- Adjusting Practices: Performance data allows for real-time adjustments. If a particular type of fertilizer is being under-utilized, data can help pinpoint the issue and guide actionable steps. Farmers become agile, adapting quickly to changing conditions rather than following outdated playbooks.
- Benchmarking: Understanding how oneâs crops compare to regional or national standards can foster healthy competition and drive improvements. Farmers can share insights with peers, developing best practices that benefit the community as a whole.
By investing time in analyzing this crop performance data, agricultural workers can prevent pitfalls and boost productivity.
Forecasting Yield with Data Insights
Forecasting yield through data insights is akin to having a crystal ball for better planning and resource allocation. This process involves predicting future crop yields based on historical data and current conditions. Here are several factors to consider:
- Historical Data: Aggregating past yield data helps to create a solid foundation for predictions. If a farmer has kept records of previous harvests, these can be analyzed to uncover correlations with weather patterns, soil conditions, or pest invasions.
- Seasonal Analysis: Using current weather forecasts, alongside historical yield data, farmers can gain insights into likely outcomes for the upcoming season. Understanding how elements like rainfall and temperature trends impact yield is key.
- Advanced Techniques: Employing technologies such as machine learning can refine yield predictions significantly. By integrating a broader set of variablesâlike moisture levels and nutrient contentâfarmers can forecast yields with greater accuracy.
"Data is not just numbers; it's the lifeblood that empowers farmers to turn uncertainty into opportunity."
The Role of Data in Soil Health Assessment
Soil health is a cornerstone of successful agricultural practices, influencing everything from crop yields to environmental sustainability. As farmers strive to produce more with less, understanding and assessing soil health through data has become increasingly vital. By leveraging data effectively, agricultural professionals can optimize their practices, making informed decisions that enhance both productivity and ecological balance.
Data-driven insights into soil health help identify deficiencies, monitor changes over time, and anticipate potential issues before they become problematic. This proactive approach not only supports immediate agricultural needs but also contributes to long-term sustainability of the land.
"Data is the soil from which great agricultural practices grow."
The importance lies not just in the collection of data but in its interpretation. Detailed analyses can lead to improvements in soil fertility, moisture retention, and nutrient levels, all critical for producing healthy crops. The intersection of data and soil health assessment thus demands careful consideration and methodical application of data principles.
Soil Sampling and Testing Data
Soil sampling is the first step in collecting data crucial for effective soil health assessment. It involves taking small samples from various locations within a field to achieve a representative overview of the soil's properties. This can be thought of as gathering puzzle pieces that, when put together, illustrate the complete picture of soil conditions.
- Frequency of Sampling: Determining how often to sample is key. Generally, itâs advisable to test soil at least every three to five years, but more frequent testing may be beneficial depending on cropping patterns and previous results.
- Sampling Techniques: There are different methods to sample soil, such as grid sampling or zone sampling. Grid sampling divides the field into equal sections, while zone sampling considers variable soil properties and previous management practices. Each has its pros and cons, making it vital to choose a method that suits the specific context of the farm.
- Lab Analysis: Once samples are taken, they are sent to a laboratory for analysis. Here, scientists assess various soil attributes like pH levels, texture, organic matter content, and nutrient availability. Each factor plays a role in plant growth, lending important insights into how soil can be managed more effectively.
Interpreting Soil Data for Management Practices
Interpreting soil data is like reading the story of the soil. The numbers presented in a lab report can seem daunting at first glance, but they can unveil secrets about the health and potential of the land. Looking closely at the data can guide agronomists and farmers in tailoring their practices to better suit the specific needs of their soil.
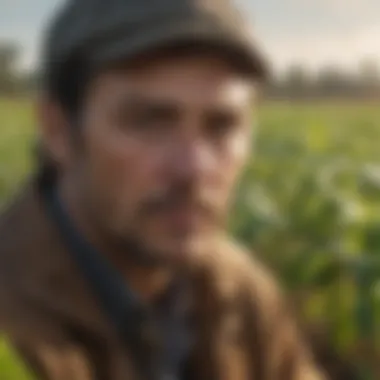
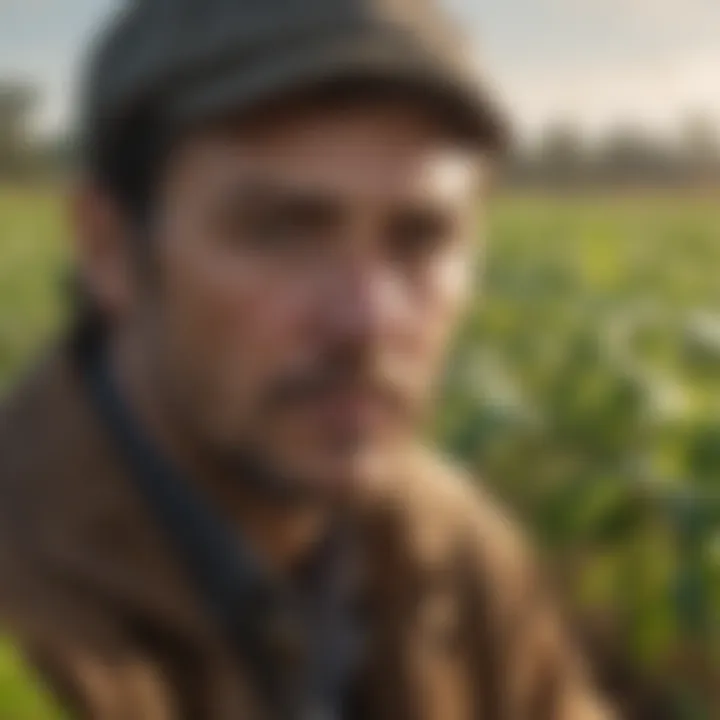
- Understanding Soil pH: Knowing the pH levels is crucial, as it affects nutrient availability. For instance, a pH below six may hinder phosphorus uptake, while a higher pH might limit iron absorption. Adjustments can then be made through the addition of lime or sulfur to achieve the ideal levels.
- Nutrient Management: Soil testing reveals deficiencies and excessesâinformation critical for applying fertilizers judiciously. For example, if data shows low nitrogen levels, targeted nitrogen applications can boost crop growth without overspending on unnecessary inputs.
- Long-term Trends: Tracking changes over time can inform sustainable practices. For instance, if data reveals a decline in organic matter, farmers might consider implementing cover crops or reduced tillage to enhance soil health. This not only aids in productivity but also supports carbon sequestration efforts, benefiting the environment.
Through a nuanced understanding of soil sampling, testing, and interpretation, agricultural professionals are empowered to make data-informed decisions that elevate their farming practices. This synergy between data and soil health not only maximizes yields but also fosters responsible stewardship of the land.
Data Utilization in Pest Control Strategies
Pest management has always been a thorn in the side for agricultural producers. The evolving challenges posed by pests demand more than just traditional methods; they require a data-driven approach. By leveraging data analytics, farmers can discern patterns that help them outsmart these nuisances before they wreak havoc on crops. This section focuses on the essential role that data utilization plays in effective pest control strategies.
Identifying Pest Patterns through Data Analysis
Recognizing pest patterns is critical to credible pest control. Farmers can use data analysis to stitch together various data points from the field, including historical pest outbreak records, weather conditions, and crop rotation schedules. By parsing through this data, they can identify trends in pest behavior, pinpointing times of peak infestation.
For instance, consider a farmer using temperature and humidity readings alongside historical pest data. If records show that a particular pest thrives during a specific humidity range, the farmer can take proactive measures to either treat the crops or prepare by implementing natural pest deterrents. Hereâs an example of how data can be organized and analyzed:
- Image Analysis: By employing imaging software, farmers can analyze leaf damage to identify specific pest types.
- Weather Patterns: Use of local weather data to predict pest migration correlating with temperature changes.
- Geographical Data: Mapping pest occurrences can spotlight specific areas within a field that are more vulnerable.
By becoming adept in data analysis, farmers not only save time but also minimize chemical use, contributing to a more sustainable agricultural practice.
Developing Targeted Pest Management Plans
Once a farmer understands pest patterns through meticulous data analysis, the next step is crafting a targeted pest management plan. Tailoring pest management strategies allows farmers to deploy resources where they are most effective, leading to better yields and healthier crops.
Two vital aspects to consider in this planning include:
- Resource Allocation: By analyzing workload and resources, farmers can determine the most effective allocation for pest management without over-burdening themselves.
- Preventative Measures: Data-driven insights enable the implementation of preventive actions, such as rotational cropping or introducing beneficial insects that target specific pests.
A targeted approach may look something like this:
- Data-Driven Decisions: Use past data to plan crop rotations that avoid pests known to affect certain crops.
- Selective Chemical Application: Armed with data, farmers can choose which parts of their fields require treatment, significantly cutting down on unnecessary pesticide use.
- Implementation of Biocontrol Agents: Understanding ecological data helps identify beneficial species that can combat harmful pests effectively.
Thus, through attentively developed management plans, pests can be kept at bay, leading to a significant increase in crop health and quality.
In summary: Harnessing the power of data in pest control strategies not only enhances yield, but fosters a healthier ecosystem in the agricultural landscape.
Future Trends in Agricultural Data Usage
The agricultural landscape is evolving rapidly, shaped by a myriad of technological advancements and data-driven methodologies. Understanding these trends is not just beneficial; it is essential for farmers and stakeholders aiming to stay ahead in an increasingly competitive and sustainability-focused market. Future trends in agricultural data usage hold the promise of improved efficiency, crop yields, and resource management. Recognizing these shifts can help practitioners leverage emerging technologies and methods to optimize their operations.
Emerging Technologies Impacting Data Practices
A range of emerging technologies is revolutionizing data practices in agriculture. These innovations are geared towards enhancing the accuracy, efficiency, and usefulness of agricultural data. Some key technologies include:
- Internet of Things (IoT): Devices connected to the internet can collect real-time data from fields, monitoring everything from moisture levels to pest populations. For instance, moisture sensors placed in fields provide instant information on soil conditions, enabling timely irrigation decisions.
- Drones and Aerial Imaging: Drones can cover large agricultural areas quickly. They collect high-resolution images and data that help in mapping fields, assessing crop health, and identifying pest infestations. This aerial surveillance allows for more focused interventions, saving time and resources.
- Blockchain Technology: By providing a secure and transparent way to record transactions, blockchain can enhance traceability in food supply chains. This technology ensures that data remains intact from farm to table, fostering consumer trust and safety.
These technologies create an ecosystem where data becomes more accessible and actionable. However, the integration of these systems requires careful planning and training for those involved in agriculture.
"Emerging technologies are reshaping agriculture, making data more actionable and transforming how farmers engage with their land and markets."
The Role of Artificial Intelligence in Data Applications
Artificial Intelligence (AI) is another game-changer in agricultural data applications. Its capabilities allow for the analysis of vast datasets that humans simply cannot process in reasonable timeframes. AI can assist in various critical areas:
- Predictive Analytics: AI algorithms can analyze historical data and identify patterns, enabling farmers to predict crop yields, anticipate pest outbreaks, and select optimal planting times. The predictions are based on past conditions and current environmental factors, making them more reliable than traditional methods.
- Precision Agriculture: By utilizing AI-driven tools, farmers can achieve higher precision in their farming practices. This includes applying fertilizers only where needed, minimizing waste and environmental impact. AI systems can systematically evaluate the unique needs of different crop sections, tailoring interventions.
- Automated Decision-Making: AI can automate certain decision-making processes, reducing the time farmers spend analyzing data. For example, some machines can adjust watering systems automatically based on data inputs from multiple sensors, ensuring optimal growth conditions without manual intervention.
As AI continues to evolve, it holds the potential to turn data into actionable insights that can significantly enhance agricultural productivity. This symbiosis between AI and agriculture represents a promising frontier, urging stakeholders to adapt to this new reality actively.
Culmination and Recommendations
In the realm of agriculture, understanding how to effectively utilize data is not merely an advantage but a necessity for fostering a sustainable and productive environment. The journey through this article highlights the crucial principles that govern data usage in farming practices, and as we draw to a close, it becomes evident that these principles cannot be overlooked if we aim to improve agricultural outputs and practices.
Summarizing Key Takeaways
Several core insights emerge from this discourse on data principles:
- Data Integrity: The cornerstone of reliable agriculture lies in ensuring data accuracy, minimizing errors throughout collection, processing, and analysis. This vigilance safeguards decision-making and boosts productivity.
- Accessibility: It's not enough to gather data; farmers and stakeholders must have access to it. Overcoming barriers to data availability is paramount. Education and infrastructure play significant roles here.
- Ethical Considerations: With data comes responsibility. Ethical usage of data, including privacy and security, ensures that trust is built among stakeholders.
- Innovative Practices: Leveraging emerging technologies like artificial intelligence allows farmers to interpret data with greater precision, ultimately leading to proactive management strategies.
These takeaways serve as a foundation for shaping a data-driven culture in agriculture and underscore the potential for positive change.
Encouraging Data-Driven Culture in Agriculture
Establishing a data-driven culture among farmers and agricultural practitioners can lead to remarkable transformations in the industry. This becomes more pressing in a world where sustainability and efficiency are paramount.
- Training and Education: Providing workshops and resources to educate farmers about the benefits and applications of data can instill confidence and curiosity. Knowledge about data management tools and techniques makes a world of difference.
- Community Engagement: Cultivating a community that encourages discussions about data and its usage helps to create a supportive environment. Local farmer groups can become platforms for sharing insights and innovations related to data application.
- Integration of Technology: Implementing simple tools like farm management software or mobile applications for data tracking can be game-changers. Encouragement to adopt technology can demystify data use and highlight its day-to-day benefits.
- Celebrating Success Stories: Highlighting case studies of farmers who successfully integrated data into their practices can inspire others. This peer-to-peer learning is invaluable and builds trust in data practices.
In summary, the conclusion dives into the essence of fostering a culture where data is respected and utilized effectively. As the industry evolves, so too must the practices surrounding data. By encouraging an environment where data-driven decisions are normalized, we pave the way for enhanced sustainability and productivity in agriculture.